2025
U.S. Debt Spiral: What the Largest Money Managers in the World Are Saying
Will the U.S. enter a Debt Spiral? We did an interactive presentation two weeks ago with some of the largest money managers in the world (the long-term "smart money"). One of the multiple choice poll questions was how high they see 30-year U.S. Treasury rates (Tbonds) reaching over the next 4-years. The answers are compiled in the graphic. Debt spirals are driven by perceptions - if investors believe there is a risk their loans won't be paid back, they demand higher interest rates to compensate them for the risk. That's what we see in the poll results.
June 23, 2025

How Mentions of Tariffs in Earning Calls Affect Companies' Share Prices
We did a simple study that counted the number of times "tariffs" were mentioned in Earnings Call Transcripts of global companies in March 2025. The share price returns of those companies in April and May were then tabulated. Those whose transcripts mentioned tariffs 30x or more fell 4% through May 31st, while those with no mentions (thousands) rose 12.5% from April 1st to May 31st. Among all stock price factors, the number of references to "tariffs" in earnings transcripts was one of the most predictive in 2025.
June 17, 2025

A New Era in Earnings Call Analysis
With advances in large language models, Earnings Call Analysis is entering a new era. LSEG MarketPsych Transcript Analytics leverages fine-tuned NLP to extract sentiment, emotion, and topical insights from corporate transcripts. See our blog on this new approach to extracting investable insights from executive (and analyst) language: https://lnkd.in/geNCjvCJ
May 28, 2025

The Anatomy of a Relief Rally
Markets just delivered a textbook “relief rally.” A 2019 study in PLOS ONE (“Relief from incidental fear evokes exuberant risk taking”) paired mild electric shocks with an investment task. When the shock threat was removed, participants’ risk appetite—and the expected value of the options they chose—jumped ~11 %. Takeaways for today’s market:• Relief fuels risk-on behavior. As macro stressors ease, investors systematically bid up risk assets. • It’s valuation, not probability, that shifts. The brain literally assigns higher worth to the same payoff once fear subsides. • Mind the half-life. If talks stall or other threats re-emerge, the reverse can happen just as fast. Behavioral finance reminds us that emotion isn’t noise around fundamentals; it re-prices them. Managing portfolios means managing the emotional cycle too.
May 12, 2025

Tariff Talk Revisited: April Sees a -12% Return Gap
In April there was a -12% share price return gap between companies that mentioned tariffs the most vs none in their earnings call transcripts (image). A month ago we posted March's results, when the gap was narrower at -7.5%: https://lnkd.in/g6iY4YRr A new type of news is moving markets. Having the right analytics makes all the difference.
May 09, 2025

Introducing Media Radar & Global Leaders Feed: Real-Time, NLP-Powered Market-Moving Insights
On Monday, we’re launching our Global Leaders Feed — a real-time stream of social and news announcements from influential U.S. cabinet members and global leaders. Why? Because they move markets.💥 Case in point:- Today at 1:18 pm ET (17:18 GMT), President Trump posted on Truth Social that reciprocal tariffs would “PAUSE.”- Within minutes, the U.S. stock market rallied in one of its largest 30-minute moves in history (see image).🔍 And yet — CNBC and other outlets couldn't identify the reason for the surge until after the bulk of the move had already happened. ⚠️ Earlier the same day, China’s retaliatory 84% tariffs were posted on Reddit and X/Twitter — a full 6 minutes before traditional financial media and 9 minutes before a low-latency Reuters ALERT.📰📉📈 Markets Are Moving Faster Than the News 📡 Introducing Media Radar:A real-time NLP-powered feed that extracts early signals from high-impact media platforms. Our feed delivers structured JSON metadata, ready to plug into quant models, signal engines, alerting systems, and portfolio risk tools. NLP Tags Provided:🔹 Entities: 20+ types (stocks, commodities, currencies...)🔹 Topics: 1,000+ (accounting, litigation, tariffs...)🔹 Events: 4,000+ (dividends-payment, factory-build...)🔹 Sentiment: Finance, ESG, commodity scores🔹 Emotions: 14 dimensions (anger, fear, optimism...)For a sample of the Global Leaders feed or a trial of Media Radar:👉 Reach out to: [email protected]👉 Or sign up here: https://lnkd.in/gtUPfc_B
April 09, 2025

How Tariff Talk Has Evolved in US Earnings Calls Over the Past 23 Years
We analyzed mentions of "tariffs" in U.S. earnings calls over the past two decades:🔹 In 23 years of data, only the Trump presidencies saw tariffs become a major boardroom topic. 🔹 During Trump I, it took time for tariff talk to ramp up. 🔹 In Trump II, we've surpassed the prior peak — and the bulk of earnings season hasn't even hit. 🔹 Mentions are now far beyond the previous peak. 👀 Market participants are clearly bracing for geopolitical and trade turbulence. 💬 This time the Trade War does not look like a negotiation tactic.
April 07, 2025
Behavioral Finance Phenomenon: The Stock Market’s Turnaround Tuesday
📉 Get Ready for Turnaround Tuesday in the Stock Market 📈 On Monday, September 15th, 2008, I was in the green room for a financial news segment, preparing to speak on behavioral finance. A CEO of a large equity investment firm was also there. As he left, he turned to me and said: “Get ready for Turnaround Tuesday!” 📊 That Tuesday turned out to be one of the biggest rallies in S&P 500 history.Curious, I looked into it further — and sure enough, the stock market has a quirky history of rallying on Tuesdays following steep Monday selloffs. It’s a known behavioral phenomenon.
April 07, 2025

Stocks Tied to Tariff Talk Are Tanking
Stocks Associated with Tariffs are Tanking We collected all U.S. earnings call transcripts from March 2025 and grouped companies into three buckets: 1️⃣ No mention of tariffs 2️⃣ Mentions tariffs 3️⃣ Mentions tariffs 30+ times in a single earnings conference call Then we looked at April stock returns… 👀 The results speak for themselves:🔵 Companies that didn’t mention tariffs had better average performance🔵 Those that talked tariffs saw worse returns🔵 And frequent tariff talkers? Down over 16% on average.
April 07, 2025

Companies Most Affected by Tariffs in the Past 24 Hours
In the past 24 hours, the companies most positively and negatively associated with "tariffs" in the media. In the image, the horizontal bars represent the average sentiment of co-mentions of the company with "tariffs" in the past 24 hours of media coverage. At least 5 co-references required to be plotted. 👍 Possible beneficiaries (or less-affected) companies include:1. BJ's Wholesale Club - inventory is largely tariff-resistant2. Philip Morris - consumers want their staples (like tobacco) regardless of price.3. Goodyear - competitors are much more affected, so Goodyear will benefit.4. Ferarri - customers are price insensitive.5. First Solar - like Goodyear, the company will benefit from high tariffs on low-cost Chinese competitors. 👎 Among losers, shipper Maersk is sinking (sorry), Best Buy's almost entirely non-US electronics stock is becoming costlier, and Puma's "cheap" shoes are no longer so.
April 03, 2025

Falling US Stock Index Sentiment is Now Approaching 2022 Lows
An update on our Feb 25th post - falling sentiment often pulls the stock market lower in a predictable fashion, especially when sentiment is dropping from a high level. US stock market sentiment is now approaching 2022 lows, but not COVID lows. Uncertainty and fear are also rising significantly. When those two emotions stabilize, prices could rebound in a "relief rally." But we've got a ways to go before that can happen.
April 03, 2025

Sentiment of Companies Associated with Tariffs: 95% Were Negative
In the past 3 months of earnings calls we averaged the sentiment of every sentence in which an analyst referenced "tariffs." Exactly 100 companies had more than 3 such tariffs references. On average, only 5% of companies were positive, while 95% were negative. The level of positivity was anemic for those five positive companies and was exceptionally negative for the remaining ninety-five. Please join our webinar April 2nd (https://lnkd.in/gy3TjFf9) or reach out for more info about the newly launched LSEG MarketPsych Transcript Analytics.
March 31, 2025

Webinar: Accelerate Alpha with LSEG & MarketPsych
If you're interested in the power of earnings calls over markets, please join our upcoming webinar on April 2nd at 3pm UK Time / 10 am ET: https://lnkd.in/gMqN-kr2
March 31, 2025

Rebellion Research Quant Event at Columbia University
Greatly enjoyed another fantastic quant event hosted by the finance rockstar Alexander Fleiss of Rebellion Research at Columbia University. So lucky to be on a panel moderated by the maestra Christina Qi with Didier Rodrigues Lopes Petros Zerfos Chris White and Yosef Zweibach. We discussed the applications of AI models in modern investment, what AI tech is building viable value-creating products, and much more. Looking forward to the next one!
March 21, 2025

How a Single Trump Social Media Post Creates or Destroys Billions in Value
The information that is moving markets is changing - now a single Truth Social post by Trump creates or destroys tens of billions in value. See the minute bars for Solana (SOL) below move after Trump's Truth Social post on the U.S. crypto reserve. Contact us for access to the market moving data you need in real-time.
March 17, 2025

Declining Sentiment on Tesla Signals a Price Slide
Is souring sentiment on Elon Musk driving Tesla shares lower? As we noted in a post over a week ago, declining sentiment on the US stock market indicated a price slide was likely (it happened). A similar effect seems to be hitting Tesla shares. Let us know if you'd like to see our whitepaper on how media sentiment can predict "risk-off" regimes, especially when sentiment falls from high levels.
March 11, 2025

US Stock Index Sentiment Drops Over the Past Week: Further Downside Expected?
Overall US stock index sentiment dropping fast over the past week. Sentiment downturns from high levels usually lead to further downside in markets (sentiment Granger-causes price momentum). Ask for our whitepaper.
February 25, 2025

Trump's Social Media Messages' Effects on Global Markets
Trump is moving global markets through his Social Media messages, in the below example, Crude Oil dropped up to 3% based on his messages about ending the Ukraine War. Let us know if you'd like more information about our low-latency news and social media data feeds.
February 13, 2025

Positive Sentiment Ranking of Companies Benefitting from Easing Tariff Concerns
Another week of Tariff discussion, with several companies on the positive side of sentiment due to relief of Tariff fears. The image shows companies associated with tariffs ranked from most to least positive over the past week, according to news and social media. Note Amazon is being said to evade the worst of tariff pain. Nucor is a U.S. steelmaker likely to benefit from 25% tariffs on non-US steel. Whaleco (Temu) got a reprieve from Trump's proposal to tax packages from China.
February 10, 2025

MarketPsych Data on WRDS - Social sentiment on meme stocks, crypto, and more
We're excited to announce that the MarketPsych Data WRDS Edition for both financial and ESG themes and sentiments are now available for research here on WRDS.The dataset is unparalleled in its global news and social media coverage. It uniquely provide sentiment, emotion, and thematic scores for every financial asset including real estate, interest rates, and cryptocurrencies. A point-in-time ESG data set is also available.SUMMARY: MarketPsych WRDS Edition (MWE) includes Buzz and Sentiment scores - derived from thousands of global news and social media sources dating back to 1998 - for dozens of topics across more than 42 sectors and industries, 275 equity indices, 170 currencies, 3,000 locations, 130,000 companies, 112 commodities, and 600 cryptocurrencies. The ESG version includes ESG Buzz and Sentiment scores for dozens of ESG topics across 130,000 companies and 250 countries and territories. Data is published separately for news and social media. MarketPsych's data is used by researchers and quants in over 25 countries. MarketPsych's data has been extensively researched in academic and industry studies and has appeared in over 300 academic papers in top finance journals, including the Journal of Finance, Energy Economics, Journal of Portfolio Management, and the Journal of Financial Economics.Please visit us on WRDS or respond to this email if you'd like more information or sample data. And please note, MarketPsych's data is also available via the London Stock Exchange Group (LSEG)).Best wishesThe MarketPsych Data Team
February 10, 2025

Ranking of Companies Most Affected by Tariffs Negatively
Companies ranked by the negativity of tariffs for their businesses, summarized from the past 7-days of media about these companies. The lowest ranked is Constellation Brands, who imports alcoholic beverages into the automotive companies occupy most of the bottom 10. There is concern about tariff blowback onto Palantir and Nvidia. METHODOLOGY: Sentences with more than 30 co-occurrences of a company name and synonyms of "Tariffs" (import taxes, etc...) in a single sentence were selected from the past 7 days. The average sentiment of those sentences was calculated and is represented in the length of the blue bar in the image below, where a longer bar indicates more negative sentiment.
February 03, 2025

Sentiment Ranking of Publicly-traded Companies Associated with DeepSeek AI
Publicly-traded companies associated with DeepSeek AI ranked from most positive to most negative portrayals by the media. On the positive side, Apple could use cheap AI in it's phones and AMD's chips can be used to create DeepSeek-like models. On the negative side, ASML (excellent AI without their extreme Ultraviolet), Oracle (just announced billions of investment in the unnecessary(?) StarGate), and Broadcom (designing for NVDA). Contact us to learn more about the power of real-time NLP for discerning market trends.
January 27, 2025

Most Positive Industry in 2024: Nanotechnology
Nanotechnology is 2025's most positively-discussed industry in global business media. In 2024 Robotics was top, and it is ranked third for 2025. See image below for the 2025 rankings and the top publicly-traded nanotech companies (ranked by mention count, not by quality - DYOD).
January 17, 2025
2024

New Research on Greenwashing Detection Combining Slow vs. Fast-moving ESG Scores
New paper on greenwashing detection by combining Slow (filings-based) vs Fast-moving (media-based) ESG scores. Blog post with paper link here: Slow and fast ESG scores: what do they tell us? Thanks to the diligent research efforts of Svetlana Borovkova and Probability & Partners. Data provided by LSEG Data & Analytics.
November 30, 2024

Ranking of Companies Based on the Average Tariff-associated Sentiment
We assessed the sentiment of all companies mentioned in media referencing tariffs in the past 3 days. We ranked the companies by their average Tariff-associated sentiment and plotted them below. A minimum of five (5) references in the past 3 days was necessary to be on this list.
There are a few surprises - some from the EV space below:1. BYD is positive because it is such a low-cost producer that even with high tariffs its cars will be relatively inexpensive in the U.S.2. Tesla is negative because of the retaliatory tariffs that China is likely to impose in any trade war, hurting Tesla's ability to sell in China plus raising the cost of cars produced at its Shanghai factory.3. Rivian is negative because its cars are already relatively expensive in the U.S. and it has a China-based supply chain, which will lead to even higher costs.
November 09, 2024

Media Sentiment Ranking of Companies Associated with Trump's Victory
The media is declaring some companies winners and losers following Trump's victory. Below are companies associated with Trump's victory and ranked by media sentiment over the past 24 hours. Note Tesla (Elon Musk) and Palantir (Peter Thiel) are most positive while Meta (Mark Zuckerberg) is most negative.________The methodology behind this study:1. Identify all companies mentioned in the same sentence with "Trump".2. Sentences were selected from 1,000+ global business and investment news and social media sources and published in the past 24 hours. 3. All companies must have been associated with Trump in the same sentence at least 50 times.4. Average the sentiment of each sentence.5. Plot them from most to least positive sentiment in a bar chart (below).
November 07, 2024

US Election 2024: Sentiment vs. Attention – What Truly Decides the Outcome?
In business media, Kamala has been associated with more positivity (positive sentiment) in the sentences that discuss her versus the sentences associated with Trump. But importantly, the volume of conversation (attention) heavily favors Trump.
So what will determine the US election outcome - sentiment or attention?
November 05, 2024

Sentiment Ranking of Companies Associated with AI Chips and GPUs
We searched our tools for the publicly-traded companies associated with "AI chips" and "GPUs", and we sorted them by media sentiment, with the most positive at the top. ASM International was the most positive over the past 90-days.
Of course, Nvidia was the most mentioned overall, but its average sentiment, although positive, did not qualify for the list.
As noted in our prior posts (and research), positive media sentiment has historically tended to lead prices higher.
October 01, 2024

Is the Trend Still Your Friend?
Dear Readers,
Today we open with one of the earliest observations of human behavior in financial markets. More than 400 years ago Josef De La Vega identified a behavioral pattern in his advice to individual investors.
“As there are so many people who cannot wait to follow the prevailing trend of opinion, I am not surprised that a small group becomes an army. [Most people] think only of doing what the others do and of following their examples. . . .” — Josef de la Vega, 1688, “Confusion de Confusiones” (the first book describing a stock market: the Amsterdam Exchange)
October 01, 2024

Effect of Volkswagen's Planned Factory Closure on Sentiment and Share Price
The image shows how VW's share price reacted after announcing their first planned closure of a German factory.
In our research we've identified a consistent 1-2 day negative return for stocks following a surprising negative news release. This average negative return is still true for both small and large stocks, including constituents of major global indices. Report coming soon.
September 10, 2024

Sentiment Shift Regarding the US Economy's "Soft Landing" Possibility
Sentiment about a "soft landing" for the U.S. economy has turned from positive ("yes, soft landing is happening") to negative ("we're going into a recession") over the past few days.
In the chart below the blue columns are the daily count of mentions of "soft landing" in the global business media. The blue line represents the daily average sentiment for the sentences where "soft landing" appears.
The soft landing sentiment flipped negative on Tuesday, Sep 3, 2024 after the ISM showed a continuing mild manufacturing contraction through August. When the narrative shifted, the equity markets started to sell-off.
September 06, 2024
The Performance of Companies Mentioning "AI" on Earnings Calls
How did companies mentioning AI in their Earnings Calls perform over the past 5 years? LSEG's Haykaz Aramyan, PhD and our own Tiago Quevedo Teodoro created a thematic portfolio using our new Transcripts Analytics. See the report here: https://lnkd.in/eNdbDpdZ
August 05, 2024
Companies Benefiting and Suffering from the CrowdStrike Outage
Companies affected by the CrowdStrike outage ranked by their outage-associated sentiment in business media.
Amazon, Zscaler, and FedEx look to benefit (note their positive associated sentiment), while Telstra, Delta Airlines, Tesla, and American Airlines are hit hardest by the software glitch.
July 19, 2024
Bitcoin’s Sudden Drop Preceded by a Decline in Social Sentiment
Bitcoin's sudden drop was preceded by a fall in social sentiment. While there may be a short-term bounce, sentiment still hasn't completely shaken out the weak hodl'ers.
July 08, 2024
Changing Trends in Apple’s Products and Services Mentions During Earnings Calls
The LSEG MarketPsych Transcripts Analytics offers insightful perspectives into the focal points of a company. The accompanying graph, developed by our colleague Elliot Chung, illustrates this by detailing the proportion of mentions that various Apple products and services received during Apple's earnings calls. This is measured as a fraction of the total mentions of all products and services. In the early 2000s, the Mac accounted for nearly 50% of these references. However, post-2007, the iPhone emerged as the predominant topic of discussion. At its peak, the iPad nearly matched the iPhone in terms of mentions, though it has since seen a decline in focus. Although the VisionPro represents one of Apple's most significant recent launches, it is currently referenced only about as frequently as the AirPods and the Apple Watch. Finally, there is a long-term trend in the overall increase in references to services (such as Apple TV, AppleCare, iCloud, etc.)
July 03, 2024
Companies Associated with NVIDIA in the Media
Which companies are likely to benefit from Nvidia's incredible revenue surge?
By scanning global news and social media over the past 30-days, we found the companies with the highest percent of co-references to the topic "Nvidia" in each sentence. In the image below, the company name is to the left and the blue bar length represents the percentage of sentences containing the company name that also contain the proper noun "Nvidia".
1. Dell supplies server hardware2. OpenAI (private) runs on Nvidia's H100 GPUs.3. Nvidia Corp is the legal entity4. Global Mofy is a declining penny stock being linked with Nvidia in social media (caveat emptor).5. Foxconn's stock rallied alongside Samsung and HK Hynix after Nvidia's blowout earnings last week.6. Astera makes components used in Nvidia GPUs.7. NetApp is partnered with Nvidia and provides software tools to manage data across Nvidia infrastructure....and many more (see image below)
May 27, 2024
Earnings Conference Call Sentiment Systematically Impacts Share Prices
Stock prices of companies with the most positive earnings conference call hashtag#sentiment outperform over the following month. Below is a monthly updated example using past 90-day deciles.
• UNIVERSE: All US companies with available transcript data• REBALANCING: Monthly at the close of the last trading day• LOOKBACK: 3 months (usually last available quarterly call)
Note the stocks are bought at the beginning of the month, up to 90 days after the earnings conference call. The price drift is quite significant and long-term.
May 20, 2024
This is the Most Positive Earnings Season Since 2002 (according to earnings call sentiment)
Better-than-expected results have appeared more frequently than not in this earnings season. LSEG (London Stock Exchange Group) Workspace data shows that about 56% of companies have beaten the mean estimate. Such strong performances have been reflected in the earnings conference calls. In the plot below, we compare the average financial sentiment (from the LSEG MarketPsych Transcript Analytics) of all available earnings calls in the quarter until today with that of the same period in previous years. This is looking to be the most positive sentiment earnings season since the start of the data in 2002. Year to date, the FTSE All-World Index is up 8%, which is above average for the same period in previous years, although not abnormally higher.
May 16, 2024
U.S. Earnings Call Sentiment Reaches a 20-year High
Over the past 20 years corporate sentiment on earning scalls has been increasing (blue line) alongside stock prices (Russell 2000, IWV, black line).
Our research on quantile performance indicates that highly positive sentiment calls precedes stock price hashtag#outperformance (a post on that effect coming soon).
But why is higher call sentiment occurring? Could it be that consistently improving corporate fundamentals are reflected in more positive call sentiment? Are analysts becoming more polite (or maybe only the friendliest are selected to ask questions)? Or is it a result of executive communication coaching to avoid gaffes and add more positive polish? Or maybe all of the above…
May 15, 2024
How High CEO Optimism Drives Stock Outperformance
Public US-based companies led by highly optimistic CEOs have outperformed their peers over the last two decades. Our preliminary analysis, using the LSEG (London Stock Exchange Group) MarketPsych Transcripts Analytics data feed, reveals that companies helmed by the top 5% of CEOs, in terms of optimism levels during Earnings Calls, exceeded their peers' future stock performance by an average of 0.5% per month. The optimism metric in the data feed is one of 13 emotional dimensions evaluated in each sentence of the earnings calls. It utilises an LLM-based architecture designed to assess the probability of speakers conveying specific emotions.
May 15, 2024
In Earnings Calls: Top 10 Most Optimistic U.S. CEOs
The LSEG MarketPsych Transcripts Analytics offers detailed sentiment analysis on corporate communications, including quarterly earnings calls. It analyses each sentence to produce insights on topics, sentiment levels, and the probability of several emotions, including optimism.
Our initial studies using this data suggest a positive correlation between CEO optimism during earnings calls and relative stock price performance in the following months. With hundreds of earnings calls for Q1 2024 already underway, we present a partial rank of the top 10 CEOs from Russell 3000 companies based on their optimism during the current earnings season. As of now, Mr. Olivier Le Peuch, CEO & Director of Schlumberger, has had the most optimistic discourse.
While Optimism is depicted in the underlying blue bars, the superimposed black bars depict the financial sentiment level of these CEOs. The optimism is higher than sentiment for every CEO. Notable disparities between the optimism and sentiment levels, such as those observed in the case of Ms. Joanna Geraghty, CEO & Director of JetBlue Airways, could also serve as valuable features for future stock price modelling.
May 08, 2024
Optimism on Full Self-driving Near Historical Peak
"And I think it might be the biggest asset value appreciation in history when that day happens when you can do unsupervised full self-driving."~Elon Musk on Tesla's earnings call yesterday.
The optimism on full self-driving is near its historical peak, as depicted in the image below.
The image shows the average sentiment sentences referencing "FSD", "self driving" and "self-driving" (red line) in Tesla's earnings calls since 2019. Tesla's stock price is in black.
April 29, 2024
Expectations of Interest Rate Moves Drive Mid-term Stock Performance
Interest Rate expectations drive U.S. stocks in an inverse relationship (rates up => stocks down). As Warren Buffett famously said, "Interest rates are to asset prices … like gravity is to the apple."
Expectations often drive financial behavior. Since the monthly CPI came in above expectations last week, some economists are now expecting the Fed to raise before they lower rates in 2024-2025.
The chart below shows the rally in the Russell 1000 (top 1000 US stocks) since Oct 2023 as expectations for rates fell. The blue shading was placed between a 90-day and a 200-day average of interest rate forecasts derived from natural language processing (NLP) on thousands of news and social media sources discussing U.S. interest rates (sentiment analysis).
Note the stock rally was inversely correlated with rates expectations since October. As the narrative shifts and forecasts for rates move higher, we could see more air coming out of stocks until more assuring CPI numbers come in.
April 22, 2024
Bitcoin Sentiment Ahead of Halving
The overall media sentiment about bitcoin is in the high end of its range. According to academic research on social media and daily asset prices, "Sentiment predicts positive next-day returns, but attention predicts negative next-day returns." https://lnkd.in/g8GgXdHX. We see a similar effect at monthly and longer horizons for many assets (stocks, currencies, etc...). News media attention to bitcoin lately has been very high.
The Bitcoin Halving is likely to occur in late April. From a psychological perspective, the increasing scarcity of bitcoin increases its attractiveness. Yet the last halving was May 11, 2020, and previously one occurred in July 2016. Before and after the past halvings, there was no clear price impact of the events.
I've noticed in recent conversations at finance events that the launch of ETFs (specifically the SEC approval) has legitimized Bitcoin in the eyes of conservative financial institutions and family offices. Many are discussing new bitcoin products and investments. Surprisingly to me, the tradfi adoption of bitcoin as a store of value seems to be in early stages.
So is Bitcoin peaking? What does the halving mean? It's hard to know in the short term: sentiment is higher (and vulnerable to a fall). The high overall attention implies downward pressure in future months. Longer term (years) the momentum is still positive.
April 10, 2024
Largest Cryptocurrencies Sentiment Heatmap: March 2024
Historically social media sentiment about individual cryptocurrencies tends to drive their prices higher. The highest sentiment large coins in March 2024 so far are #DOT #ARBITRUM #MATIC #AVAX #BNB.
March 13, 2024
Top Small Cryptocurrencies by Sentiment
Here's a ranked view on some of the smaller #cryptocurrencies' sentiment from #socialmedia covering the period March 1 through March 12, 2024.
March 13, 2024
Our Media-based Stock Prediction Model, Continued Outperformance
We built a 30-day U.S. stock predictive model based on Media Sentiment using a simple Machine Learning algorithm - updated returns in the below image. Humans, even in hyper-competitive environments like the US stock market, respond predictably to news and social media content.The model was put in production in Aug 2019 and launched commercially on Jan 2020 in partnership with the StarMine team at LSEG Data & Analytics.The model ranks stocks (1 to 100) based on their likelihood of outperformance using a ML algo applied to only our LSEG MarketPsych Analytics news and social media-derived data. The blue line in the chart below depicts the spread between the top-ranked decile (10%) of stocks and the bottom-ranked decile - the absolute return portfolio. The gray-shaded regions are the 2-year training periods.The model performs similarly regardless of market cap. It is orthogonal to other StarMine models. It has about 30% monthly turnover, with no transaction costs assumed. Longer term models also work well with media data (we've tested out to 3 year forecasts).
March 12, 2024
Post Office Ltd and Fujitsu Scandal: Impact on ESG Scores
For our UK readers, the evolving scandal at the UK's Post Office Ltd & Fujitsu is rapidly impacting Fujitsu's ESG scores.
Background: The digital accounting system that the postmasters (regular people) entered their daily sales & cost calculations into were being fraudulently altered via remote access from the UK's Post Office Ltd / Fujitsu.
In our ESG data Post Office Ltd has the lowest (1st percentile) Community & Social scores and a 4th percentile ESGControversies score (very bad).
The blame has gradually shifted from Post Office Ltd to Fujitsu over the past month in social media, and Fujitsu's ESG scores have dropped substantially - see graphic below.
February 19, 2024
Top Robotic Initiatives Companies
In our earlier post we identified #Robotics as the industry with the most positive media sentiment in 2023 (and 2022).Attached is a list of the companies most associated with Robotic initiatives or products in the past two months. Based on our #quant research, the valuations of such companies tend to (on average) outperform when associated with higher media sentiment (as they have been).
January 02, 2024
Positive Industries in 2023
Every year we look at the most positively viewed industries in global #businessnews and #socialmedia.For 2023 we see #Robotics on top (as it was in 2022) followed by #RenewableEnergy, #Tourism, #Fintech, and #Infrastructure.Further down the list the industries with a country code after them indicate the industry is primarily favored in one region (US = United States, CN = China).The stock prices of the highest #sentiment companies and industries on average outperform over the following year.In 2022 AI was #2 but fell to #33 based on legal and social challenges.We wish you all a happy, healthy, and prosperous 2024! #happynewyear
January 01, 2024
2023
Bitcoin Momentum
#Bitcoin sentiment has been at highs and the bull keeps running - a phenomenon we call sentiment momentum. In this paper https://lnkd.in/gasgp9BQ we show that across thousands of global stocks, sentiment precedes price momentum (using Granger Causality Analysis), and the chart below illlustrates that effect in #BTC.In the chart, the black price bars are BTC/USD and the smooth lines are two moving averages of #sentiment (30 day and 90 day superimposed). The red shading indicates negative sentiment pressure (the 30-day sentiment is lower than 90-day), and blue shading indicates more positive sentiment.Social sentiment on BTC is the highest since 2020, and news sentiment is the highest since 2018. When sentiment falls substantially, we're likely to see a price correction, but that isn't happening yet.
December 11, 2023
Weight Loss Drugs Sentiment
#Antidiabetes drugs (#glp1 agonists) have been in the news not only for success in treating diabetes, but also for the shortages caused by off-label uses for #weightloss, #addictivedisorders, and #longetivity.#MorganStanley sees the drugs decreasing food industry profits and dramatically impacting stock prices, with an 80% gap emerging since Jan 2023 between stocks of companies benefiting from these medicines (largely #pharma) vs those being impacted by these medicines (retail and fast food companies). Here is a #foodindustry perspective: https://lnkd.in/g5smxCGj. Big picture, these medicines may substantially reshape US society.We get a lot of questions about which of these medicines is winning in the public sentiment space, and our analysis shows #Zepbound (daily green line below) with higher media sentiment than #Ozempic (blue line below). Zepbound was the last GLP1 medicine to be released, about 10 days ago. We'll post more as the situation evolves...
November 28, 2023
Investment Opportunities using Emotions paper
This recent academic paper - Investment Opportunities Offered by Investor Emotions - (https://lnkd.in/gTg4F9qu) implements a practical portfolio construction strategy built on existing research that identify investor emotions as precipitative of post-earnings excess returns.Even accounting for steep transaction costs, the authors demonstrate a long-short strategy yielding an 18% annualized return that has low or insignificant correlations to all FF5 factors using #Refinitiv #MarketPsych data.
October 20, 2023
Portfolio Allocation using RMA
A recent paper in the #JournalofFinance investigates how investors approach their portfolio allocation. The authors find evidence for an investor heuristic called naive buying diversification (NBD), where investors allocate 1/N of their money in the N assets bought on that day (instead of considering their portfolio composition as a whole). The authors used our MarketPsych Analytics ("TRMI" in the paper) to test an explanatory hypothesis for such behavior. Their analysis provides support for a stock-picking explanation: NBD is more common when investors buy similar stocks, such as stocks with similar buzz (amount of media chatter). The findings could "have implications for the design of contribution-based saving plans, such as pension schemes". https://lnkd.in/da7m7BSdThe researchers combined datasets of #Barclays brokerage transactions (and demographic data), LSEG (London Stock Exchange Group) price data, and #MarketPsych news data.The below plot shows the function by which investors are more biased to invest in the similar buzz stocks. Lower buzz difference between two stocks (one proxy for similarity) is correlated with higher likelihood of them being purchased using the NBD pattern.
October 13, 2023
China Residential Property
#China's 3rd largest residential real estate developer #CountryGardenHoldings Co Ltd defaulted on its bonds this month. Note that our #Gloom score for the company was already triggering well in advance (image attached).The past two weeks distress in the Chinese financial system are creating a #RiskOff attitude in global markets.Per #Reuters: "China's property sector ... is the largest asset class in the world, with an estimated market value of around $62 trillion... The next thing to watch is how regional governments, many of which rely on real estate revenue, manage their debt."On top of commercial (and multifamily) real estate distress in the US, we may see a #HardLanding from #MonetaryTightening after all. (Markets have been betting on a soft landing until this week).
August 23, 2023
AI Trading: What Could Possibly Go Wrong...
The popular press is abuzz with articles about AI's transformation of investing, financial research and analysis, and stock market forecasting. At MarketPsych we work in the field of AI with large language models (LLMs) including chatGPT, and in today’s newsletter we explain some LLM trading basics as well as our own successful AI agent designed for stock price forecasting. To illustrate the value of AI in investing, we'll start with the human weaknesses it is designed to exploit…
Investing on fear
AI vs AGI
chatGPT is a non-sentient model (it's not conscious), and there is a big gap between it and the type of artificial general intelligence (AGI) described in the Fear Index. As physicist David Deutsch noted: “AI [artificial intelligence] has nothing to do with AGI [artificial general intelligence]. It’s a completely different technology, and it is in many ways the opposite of AGI … An AGI can do anything, whereas an AI can only do the narrow thing that it’s supposed to do.”
July 16, 2023
Russia's regime change score: minute-to-minute
Below is a minutely depiction of our #RegimeChange score (blue line) for #Russia from June 22nd to 28th, 2023 UTC time.MarketPsych's real-time NLP engine scours macroeconomic themes, political risk, and country-level signals around 100' of risk-related events from 1000s of #globalnews and #socialmedia sources in 13 languages. In partnership with Refinitiv, an LSEG business our clients set risk management alerts for 100s of such risk-related events. Available country-level indicators include #SocialUnrest, Terrorism, InflationForecast, BondStress, and 100+ more.
June 28, 2023
Stock-forecasting AI model shows impressive performance
In Jan 2020 our MarketPsych team launched a stock predictive model in partnership with #StarMine and Refinitiv, an LSEG business, called the MarketPsych Media Sentiment (MMS) model. It is an #AI based model, making stock forecasts based solely on media themes and #sentimentanalysis.The model was approved for production in Aug 2019 (red dotted line in the image) and has been sold commercially since Jan 2020. It showed yet another great performance in May 2023.
This model ranks stocks daily according to their expected relative performance for the following 30 days. This past May, the spread between the highest and lowest-ranked US stocks was 4.3%, accruing to +13.4% on a 12-trailing months basis. Among large-caps, the performance is at an impressive +22% with a Sharpe of 3.3. The decile spread is plotted in the image as the blue line.There are predictive patterns embedded in the emotions and themes expressed about companies in news and social media. #behavioralfinance #quantitativefinance #quantitativeanalysisFor an introduction to MMS, please watch the promo video at: https://lnkd.in/gNqwX7fx
June 21, 2023
U.S. commercial real estate sentiment plummeting - time to buy?
With U.S. commercial real estate sentiment plummeting, is it time to buy?In the chart below we plotted the #Russell1000 price versus two moving averages of media-derived U.S. #commercialrealestate sentiment from 2020 to now. When the 90-day is under the 200-day, the gap between the lines is shaded pink. Note that COVID led to a slight downturn due to WFH (work from home), then there was optimism about RTO (return to office), but today's interest rate / hybrid work habits-driven collapse depressed sentiment much more.The Wall Street Journal last week reported distressed asset buyers buying properties like #AgaveHoldings. Per managing director Jose Perez “We have learned from the past, that when everyone is selling, buy; and when everyone is buying, sell.” https://lnkd.in/gpqKujd9#sentimentanalysis #sentiment #behavioralfinance
May 20, 2023
US Inflation to Drop to 4.3% Next Month: Insights from CPI and Predictive Model
The U.S. CPI came out yesterday showing inflation at 4.9%. Our predictive model, based on the aggregate inflation forecasts from news and social media, shows a further significant inflation drop over the next month to 4.3%. This model has consistently had a 30% tighter fit to the U.S. CPI than the consensus of experts since it began rolling-forward (expanding window architecture) in 2004.Once interest rate expectations plateau, then the market may resume its upwards drift.
May 11, 2023
Schwab Bucks Trend in Latest Bankrun as Non-US Banks Take a Hit
Today's bankrun is targeting large non-US banks: Credit Suisse (a repeat target from back in October 2022) and BNP. Note that Schwab is in the green, probably due to being in the US (Fed backstop) and having a supportive CEO aggressively buying his own shares.
We plotted the overnight bullishness in the media about select major banks, below:
March 15, 2023
Media Mentions over SVB: Other Regional Banks and Tech Companies Take Center Stage
These are the companies most frequently mentioned in the media along with SVB in the last 5 days. Many are large financial institutions (LFIs, gray bars) that were mentioned due to stock prices falling across the board. However, the main concern surrounding SVB is other regional banks (blue bars), such as First Republic, Signature Bank, and Pac West Bancorp.
Several technology companies have also been frequently mentioned due to their possible exposure to SVB (red bars): Roku, Circle, Roblox, BlockFi, Coinbase, and Etsy
.
March 12, 2023
Sometimes News Sentiment Drives the Market, Sometimes It is Only Reacting
According to a new paper, news sentiment sometimes drives the stock market, and sometimes reacts to it. Specifically, news drives market prices during periods of upheaval (debt crises, Brexit and Covid-19 to name a few). Sentiment reports on market action - after the fact - during less volatile times.
Spillover between investor sentiment and volatility: The role of social media by Ni Yang (Auckland University of Technology), Adrian Fernandez-Perez (Auckland University of Technology) and Ivan Indriawan (University of Adelaide)
March 03, 2023
Risk Signals for Norfolk Southern's Train Derailment
Following on #Adani, another example of real-time #AI-detected ESG controversies monitoring capturing events as they evolve. Note how the controversies score decline leads the share price lower.
We send our sympathies to the residents of East Palestine, OH.
February 28, 2023
Adani: AI Detects Investment Risk Faster Than Humans
After Hindenburg Research released their report highlighting AdaniGroup's accounting misdeeds, our ESG scores immediately signaled danger, yet the stock hovered for a day as investors entered a state of denial (see the quotes from representative social posts below).
In the chart below several Adani long-term ESG Controversy scores are plotted against the share price of Adani Total Gas (the biggest hit).
Our 365-day average ESG Controversies scores fell for all Adani companies, and Adani’s share prices started to plummet the day after. (Our 60-second scores were even faster...)
February 27, 2023
Americanas SA One-day Decline, the Largest in Recent Memory in the Ibovespa
The CEO and CFO of Americanas SA resigned on Jan 11th due to Accounting inconsistencies at the company.The stock posted the largest one-day decline of any of the 89 companies currently in the Ibovespa index in at least 20 years. AI-based ESG analytics picked up the drama as it happened.Hiring new CEO Sergio Rial (formerly CEO of Santander Brazil) on August 20, 2022 was a good public relations move for Americanas.The company’s ESG Management score (our real-time ESG metric, green line) jumped after the announcement in the chart below (stock price is blue bars).Then on Jan 11th, 2023 (shortly after assuming his new job on Jan 1st) he reported accounting irregularities, causing both the drop in our Management score (in the final days of the chart) and the departure of the new CEO.
January 19, 2023
Top 4 US industries in 2022 News and Social Media Sentiment
We reviewed public sentiment about 40 US industries over the past year, and our data shows the most overall excitement about the top 4 below (top 10%), in descending order:
January 15, 2023
2022
Argentina's World Cup Win Shows How Major Sports Victories Impact the Local Stock Market
Note the spike higher in Argentina’s Stock Market the Merval following their historic World Cup victory last weekend.
This is similar to academic research such as “Sports Sentiment and Stock Returns” published in the Journal of Finance by Alex Edmans, Diego Garcia, Øyvind Norli - losses dampen moods and victories lift them - leading to national economic effects...
December 22, 2022
Optimism in China after Covid-Zero Policy Rollback
Note how optimism about the Chinese Stock Market rocketed following rumors (and then reality) of Covid Zero policy roll back.
Behavioral finance research shows that relief from financial fear leads to - unexpectedly - excessive risk-taking, accounting for this “COVID relief rally.”
The optimism chart was developed using a z-score of our Chinese stock market optimism index, derived from millions of news and social media comments in 13 languages, over the course of 2022.
December 16, 2022
UK Leadership Media-based Perception
Our Leadership score for the UK tracks news and social media-based perceptions of British leadership quality over time using NLP and Fall 2022 is a rollercoaster (see chart).
Most recently, the increasing cost of living is impacting Leadership perceptions.
December 13, 2022
The Economy Grows, Recession in Sight
While the economy seems to still be growing, our media-based recession indicator says it will soon be in recession.
Note that when the media begins to beat the drum on an impending recession, a self-fulfilling prophecy may kick in as businesses hunker down in anticipation of the event. In this chart we show in blue the net references to "economic growth" vs "economic contraction".
When the references to Growth vs Contraction fall below 1 standard deviation of their historical average, this has always preceded an actual recession since at least 1968 in the U.S.
November 13, 2022
Media Data as Predictor of Real Estate Prices
A depiction of U.S. real estate sentiment since Jan 2019.
The blue bars are the Russell 1000 stock index. The purple line is average commercial real estate sentiment, which is higher now that workers are going back to the office post-COVID. The green line is residential real estate sentiment, which is as low as it has been since 2011 due to rising mortgage rates.
Sentiment is measured in the U.S. business news and social media from references to each asset class.
November 09, 2022
The Acceleration of Everything
“We are not makers of history. We are made by history.”
~ Martin Luther King, Jr.
2021 was the Great Acceleration of technology and society: Memes, Anti-aging, mRNA, CRISPR, AI, Sustainable Finance, the Electricity Economy, Metaverse, DeFi, NFTs, Flying cars, and to cap it all off – surprise! – inflation.
As investors it helps to know the dominant narratives driving the market, and where we are in each cycle. In 2021 media narratives dominated the conversation and passed on silently in COVID-like waves. The contagiousness of memes, and the bold meaninglessness of many, imbued 2021’s financial markets with a beautiful insanity.
An article summarizing a few of the year’s memetic winners is here. The pace of change will slow (some mini-bubbles are popping), but markets will be forever changed. It’s an amazing time to be alive, with the Acceleration of Everything.
January 16, 2022
2021
UFOs, the Unexplainable, and Solving Mysteries with Data
For generations, U.F.O.s have been in the purview of late-night call-in radio shows and supermarket tabloids, not the Department of Defense. Now the government is publicly acknowledging that mysterious sightings can no longer be dismissed, and a major report is due in June.
~ “Are U.F.O.s a National-Security Threat?” April 30, 2021. David Remnick. The New Yorker Radio Hour.
In December 1998, on a dark snowy road in southern Sweden, I glanced above the trees and saw a small glowing oval object moving in parallel to our car. The road was straight, the sky was cloudy and black.
I pointed it out to my girlfriend, who was driving. We stared at it silently for about 10 seconds. The object moved in front of the car, hovering over the road a few hundred meters ahead. It maneuvered in perfectly straight lines – vertical then horizontal, far then near, side to side. I looked around for an explanation – was it a reflected dashboard light, a helicopter?
After a minute of silence I reluctantly asked, “Are you seeing this? What is it?”
She nodded but stayed silent.
I offered, “Is that what they call ... um ... a UFO?”
She peered at it thoughtfully, shrugged, “Mmmm, yeah, I guess so ...”
After another minute of hovering and a few perfectly straight sideways and vertical movements, and it darted away. I filed it under "Head Scratchers."
June 04, 2021
Being Greedy, Doing Good - The New Economics
As a young investor in the late 1990's the conventional wisdom was that "sin stocks" (gambling, alcohol, and weapons stocks) outperformed others. Investors could choose to either be greedy and profit or be principled and underperform. The narrative fit the transactional "greed is good" nature of the times. But it turns out the story isn't so simple.
Inflation spotting, meme stocks (see our Jan newsletter), cryptocurrencies, and the green transformation – lots happening in markets. Today we focus on the latter.
With a our new ESG dataset, we see that the performance of ESG investments is more often the opposite of the conventional wisdom - invesors can both do good AND outperform.
BALI
March 12, 2021
The Revolution Will Be Game-ified
This week's GameStop battle was the latest in a smoldering revolution - a revolution embodied in a cute Shiba Inu (and a few rainbow ponies).
Dogecoin. wow
Dogecoin is a cryptocurrency started as a joke, and it is worth $6.7 billion (as of Jan 29, 2021).
Dogecoin is a meme that resonates widely perhaps because it has no “fundamental” value. It’s human - or canine - whatever. The point is, it isn’t useful. It has no tangible value, yet it has grown to a market cap of $6.7 billion.
January 30, 2021
2020
Election Psychology & Markets
“Prepare for the unknown by studying how others in the past have coped with the unforeseeable and the unpredictable.”
~ George S. Patton
The chart above displays the S&P 500 average versus the level of media uncertainty around past (and present) U.S. presidential elections. The yellow line is the average news and social media uncertainty level. The uncertainty average includes all presidential elections back to 2000. The white dashed line is the average S&P 500 return around all presidential elections since 1928. In the longer lower plot, the red line shows our 2020 level of U.S. uncertainty coming into the Trump-Biden match-up.
In 2020, uncertainty is higher than any other election. The “uncertainty collapse” (decline in the yellow line) starting 2-3 weeks before the elections (1) correlates with a rally in the S&P 500 and (2) happens when the media (and others) believe they know the likely outcome.
October 21, 2020
Elites and Inequality
WILL THE ELITES ACCOMMODATE?
“Inclusive economic and political institutions do not emerge by themselves. They are often the outcome of significant conflict between elites resisting economic growth and political change and those wishing to limit the economic and political power of existing elites.”
― Daron Acemoğlu, Why Nations Fail: The Origins of Power, Prosperity, and Poverty
After the global financial crisis, many people lost trust in the financial system. The Fed came to the rescue, and market prices recovered. Yet the sense of economic unfairness remained. Rising asset prices helped those with assets, while wages stagnated.
June 05, 2020
Unraveling Social Trust & the Path Forward
“And with economic and cultural indexes down, with the world turned darker and more predatory, we will go through more. We thought we’d be telling our grandchildren about the spring of 2020. Actually we’ll be telling them about the coming 10 years, and how we tried to turn everything around.”
~ Peggy Noonan, "On Some Things, Americans Can Agree". June 4, 2020. Wall Street Journal.
Last week my 11-year old daughter cried as she told our family how young neighborhood boys her age - strangers - had attacked her. They pelted her with batteries and tried to drive her off the bike path as she pedaled away from school, ramming her bike with their own.
Her mother and I explained that maybe the boys’ home lives aren’t positive. Maybe they are being raised in abusive households. But that didn’t satisfy her sense of injustice.
Her school is in an immigrant neighborhood of Amsterdam, near public housing. Here in the Netherlands there is a higher proportion of Surinamese immigrants in public housing. These are largely descendants of African slaves from Dutch Suriname. Racial issues were in the back of our minds.
June 05, 2020
Coronavirus & the Information Cycle in Markets
It's not easy training children to say "please" and "thank you" when appropriate (I must have reminded mine 10,000 times), and one unexpected benefit of the coronavirus pandemic is that my children now have a healthy hand-washing habit. Nothing focuses the mind like contagious disease.
Today's newsletter focuses on information contagion - how information infects markets, provokes denial and fear, and creates investable patterns during negative events like disease outbreaks. A PDF presentation with graphics and more information underlying the newsletter is available here.
For excellent alternative perspectives, see This New York times review projecting the disease burden of the pandemic. This James Mackintosh article in the Wall Street Journal discusses financial markets scenarios (e.g., L-U-or V-shaped bottom).
No one can deny the epidemic anymore - it's time to wash our hands and dig in.
UNDERREACTION (a.k.a. DENIAL)
I've given webinars and conference presentations on coronavirus over the past month (here's one), and I've been surprised by the wide range of attitudes towards the pandemic in different countries. As recently as last Friday I was on full flights in Scandinavia and occasionally shaking hands during meetings (and then nervously stuffing my hand in my pockets so I wouldn't touch my face).
However, at some banks in Stockholm meetings were canceled to prevent outsiders from bringing the virus into the firms. In other firms, in order to keep trading operations normal through the pandemic, employees were sorted into Team A and Team B alternating weekly home/work shifts. The general consensus in my meetings was that EU and US governments weren't taking the epidemic seriously enough. They were in DENIAL. As they say...
“Denial ain’t just a river in Egypt.”
~ Provenance unclear, but formalized by self-help guru Stuart Smalley (played by Al Franken), 1991.
March 13, 2020
Epidemics of Fear - The Cycle of Panicked Overreaction and Bounce
Eleven years ago we were trading through the Swine Flu epidemic. At the time, while I was trading our hedge fund (MarketPsy Long-Short Fund LP), my colleague Frank Murtha penned this humorous advice to relax about the outbreak (perhaps directed at me!)
Our fund's strategy took advantage of fear-driven dislocations, and we created the below image of an actual trade for our investors to understand how the fund timed investor overreaction. (In 2009 trading on social media was seen as seriously goofy, to put it politely, and we needed clear explanations of how our quant systems identified good trades).
February 04, 2020
2019
Emotional Manipulation in Markets
“All the incentives point to continuing this sort of self-extraction….Why would we stop scooping the attention out of ourselves, destroying democracy, and debasing our mental health when that’s the thing that makes the most money and Wall Street’s not going to stop funding it?”
Tristan Harris. Sep 19, 2019. “Fighting Skynet and Firewalling Attention.” Tim Ferris podcast, Minute 1:11.
"... I see people I have known my whole life slip away from me on social media, reposting conspiracies from sources I have never heard of, some sort of internet undercurrent pulling whole families apart, as if we never really knew each other, as if the algorithms know more about us than we do, as if we are becoming subsets of our own data, which is rearranging our relations and identities with its own logic, or in the cause of someone else's interests we can't even see ..."
Peter Pomerantsev. (2019). "This is Not Propaganda: Adventures in the War Against Reality."
“In the Banda Islands, ten pounds of nutmeg cost less than one English penny. In London, that same spice sold for more than £2.10s. – a mark-up of a staggering 60,000 per cent. A small sackful was enough to set a man up for life, buying him a gabled dwelling in Holborn and a servant to attend to his needs”
― Giles Milton, Nathaniel's Nutmeg: How One Man's Courage Changed the Course of History
"You need to build a fairytale that will be common to all of them.... The disparate groups needed to be unified around a central emotion, a feeling powerful enough to unite them yet vague enough mean anything to anyone."
Peter Pomerantsev. (2019). "This is Not Propaganda: Adventures in the War Against Reality"
December 08, 2019
Behavioral Manipulation & Tech - the Reckoning
“Facebook has learned how to manipulate empathy and attachment in order to increase engagement and make billions.”
~ Jennifer Szalai. Jan. 16, 2019. New York Times. "O.K., Google: How Much Money Have I Made for You Today?"
“Instead of mining the natural landscape, surveillance capitalists extract their raw material from human experience.”
~ Jennifer Szalai. Jan. 16, 2019. New York Times. "O.K., Google: How Much Money Have I Made for You Today?"
“You’re not technically the product, she explains over the course of several hundred tense pages, because you’re something even more degrading: an input for the real product, predictions about your future sold to the highest bidder so that this future can be altered.”
~ Sam Biddle. Feb 2, 2019. “A Fundamentally Illegitimate Choice”: Shoshana Zuboff On The Age Of Surveillance Capitalism." The Intercept.
April 11, 2019
2018
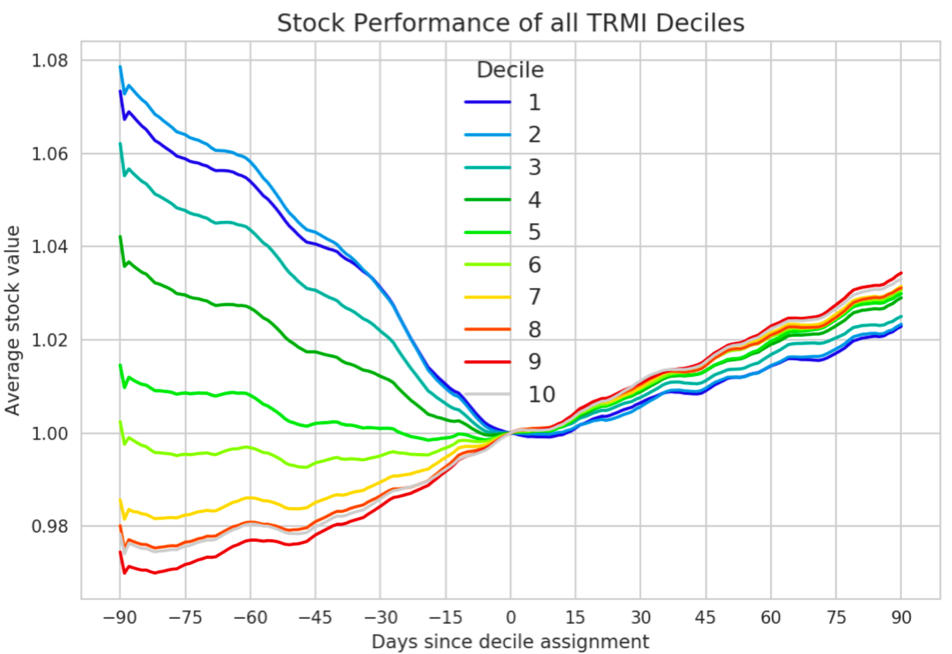
Stock Market Rout, Playing the Emotional Game, & Sentiment Feedback Loops
True story: In the first few days of October 2018 my friend Nate asked me over coffee, “Do you think we’re at the top of the market?” Over the course of an hour Nate and I went through the market prognosis in detail. Interest rates were up, housing prices falling, increasing economic uncertainty, and our sentiment indicators were all negative. We both agreed it was the top. We closed our conversation with a mutual reminder - “Time to sell everything.” A few days later the S&P 500 dropped more than 3%, beginning the rout that continues through this week.
But to be clear - I'm no hero. I didn't sell anything.
And I’m not alone in my inertia. Despite what we KNOW to be true intellectually, taking action based on that knowledge is a different beast.
Today’s newsletter examines why it’s difficult for many people to sell at tops and why - based on longer term patterns - the selling may not be over.
IT'S NOT AN INTELLECTUAL GAME
I have various excuses for my inaction in early October - I’m not a market timer, I'm not currently managing money for others, it's not worth my time and attention, etc... All seemed true at the time, yet all these excuses are intellectualizations. I could have saved a pretty paper penny getting out back then. The leap from armchair investor to professional crosses that motivational chasm.
December 21, 2018
Sentiment, the "Big One", and Bali
A few months ago my wife and I moved our kids from California to Bali, Indonesia for the year. We've been surprised by the strength and frequency of the recent earthquakes. During one earthquake I watched waves of water slosh out of our pool. During another I paused a business call as my roof groaned and light fixtures jangled. During a few late night earthquakes I’ve been shaken awake in a daze wondering, “Why is my wife jumping on the bed so hard?” Is she doing jumping jacks?!" (She had similarly foggy and illogical thoughts about me).
We have chosen to live in the “Ring of Fire” where earthquakes and volcanic activity are a fact of life – part of what makes the landscape beautiful, lush, and dramatic. And despite the instability here, we feel very lucky – residents of the Indonesian islands Lombok and Sulawesi have lost much more than pool water.
Financial markets have their own quakes and tremors, including one this week. Intellectually, we know such events will happen. But after each many people wonder “Could this be the Big One?” As a seismologist of the human psyche, in today's newsletter I feel some responsibility to explain what we know about predicting market downturns. Today’s newsletter explores market quakes: their psychological predictors, the potential this week's tremor was a precursor to the “Big One”, and a Balinese understanding of duality.
Sentiment Momentum
October 14, 2018
Profiting from Media Outrage
Don't trade angry. Seriously. If you're considering an investing decision and you find yourself feeling angry, stop, walk away, and go do something less destructive, like headbutt your bathroom wall or drop a cast iron skillet on your foot.*
~ Frank Murtha, PhD from The Seven Deadly Sins of Investing: Anger.
The popular TV show "Billions" follows the conflict between powerful U.S. attorney Chuck Rhoades and hedge fund manager Bobby Axelrod. Chuck's wife Wendy Rhoades is a psychiatrist and the performance coach for Axelrod's hedge fund, Axe Capital. In the premiere of season 3, Axelrod's professional and personal lives are threatened by rage-fueled misjudgments. During a coaching session, Rhoades performs an exercise to help him transform his anger.
Axelrod (A) notes that the seeds of his anger were planted when he - a poor kid - was bullied by rich kids:
A: "That rage grew in me. Worked like jet fuel for years." ...
R: "It was useful."
A: "Yeah it was, got me here."
R: "We can agree your rage worked for a time. I think your brain, instincts, market feel, all that had more to do with it. But for the moment let's agree that the rage worked for a time. But let's look for a moment when it was the wrong tool, and it cost you more than it made you. And about how when you allow your anger to make you blind to consequences it shuts off those more crucial skills." [my bold]
As Dr. Rhoades alluded, anger distorts judgment. Anger is a unique emotion in that it increases both risk aversion and confidence. Irate investors are less willing to invest in a socially scorned company, they feel more certain that their decision to avoid them is sound, and they hold on to these risk averse feelings even after the company has taken action to remedy the situation. As the Buddha noted, "We are not punished for our anger. We are punished by our anger." Sometimes the anger is projected onto a target, like a company, and we miss opportunities due to our anger-distorted judgment.
In today's newsletter we demonstrate powerful evidence that the stocks of companies described with anger in the media outperform their peers substantially over the following year. When a company runs afoul of the social consensus - BP due to the Gulf of Mexico oil spill, Facebook due to privacy concerns, VW due to falsifying pollution tests - investors have an opportunity.
The media demonstrates a frequently shifting spotlight of moral outrage. The phenomenon was captured in this Slate article detailing the daily social and media outrage explosions of 2014. Such social scorn often lands on publicly traded companies, hitting their stock prices. Because of the cognitive distortions associated with anger, causing investors to become risk averse, these media pile-ons provide a historically consistent and significant opportunity.
The below equity curve is one of our new quant results from our new Thomson Reuters MarketPsych Indices version 3.0 data set in the talented hands of our Head of Research CJ Liu and Alice Fu.
May 20, 2018
The Rise, Fall, & Resurrection(?) of Cryptocurrencies
The universe of numbers that represents the global economy. Millions of hands at work, billions of minds. A vast network, screaming with life. An organism. A natural organism. My hypothesis: Within the stock market, there is a pattern as well... Right in front of me... hiding behind the numbers. Always has been.
~ Max Cohen in Pi (1998, movie), Artisan Entertainment.
Restate my assumptions: One, Mathematics is the language of nature. Two, Everything around us can be represented and understood through numbers. Three: If you graph the numbers of any system, patterns emerge. Therefore, there are patterns everywhere in nature. Evidence: The cycling of disease epidemics;the wax and wane of caribou populations; sun spot cycles; the rise and fall of the Nile. So, what about the stock market?
~ Max Cohen in Pi (1998, movie), Artisan Entertainment.
My new Hypothesis: If we're built from Spirals while living in a giant Spiral, then is it possible that everything we put our hands to is infused with the Spiral?
~ Max Cohen in Pi (1998, movie), Artisan Entertainment.
“When I was a little kid my mother told me not to stare into the sun, so once when I was six, I did. At first the brightness was overwhelming, but I had seen that before. I kept looking, forcing myself not to blink, and then the brightness began to dissolve. My pupils shrunk to pinholes and everything came into focus and for a moment I understood.”
~ Max Cohen in Pi (1998, movie), Artisan Entertainment.
April 06, 2018
2017
2017's Most Trusted Financial Firms, Most Loved Retailers, and Most Innovative Tech Companies
The social media herd can turn on a dime, praising a company one month and then trashing its reputation the next (see Papa John's, below). The past year has seen many such ups and downs, and this article looks at a few of those the herd ranked highest.
MarketPsych's patented natural language processing engine quantifies emotions and themes expressed in relation to a universe of over 12,000 global companies, 45 currencies, 187 countries, and 36 commodities. The software scans articles and posts in over 800 financial social media sources and 2,000 premium news sites. The Thomson Reuters MarketPsych Indices (TRMI) data feed results from this analysis. The TRMI is utilized by the world's top funds, banks, and government agencies for asset allocation and investment research.
Using the TRMI data, we performed a year-end analysis to ascertain the top global companies as described in financial social media. Of a pool of 12,000 global companies tracked, we ranked only those mentioned on average more than 10 times daily in social media. We then ranked and identified the Most Trusted financial firms, the Most Loved retailers (consumer cyclicals), and the Most Innovative technology companies.
While global real estate and infrastructure investment firm Brookfield Asset Management was surpassed this year as the largest global real estate investor, the company remained the most widely trusted and admired financial stock by investors commenting in social media.
December 28, 2017
Bitcoin, Cryptocurrency, and the Greatest Bubble in History
"The prospects for Bitcoin are bright"
~ MarketPsych's April 1, 2013 Newsletter
"Their conversion value to national currencies depends entirely on supply, demand, security, confidence, and the whims of the investing crowd and businesses who accept them."
~ MarketPsych's April 1, 2013 newsletter
"Keep in mind that most people feel inertia ... we often wait to make the obvious investment after it has been proven valuable (and we are too late in entering)."
~ MarketPsych's April 1, 2013 newsletter
"Ultimately, cryptocurrencies are just digital assets native to a specific blockchain"
~ Josh Nussbaum. Oct 16, 2017. "Mapping the blockchain project ecosystem." Techcrunch.
December 03, 2017
The Last Supper, Sentiment Cycles, and Human Skulls
One of you shall betray me.
~ Jesus, speaking to his disciples the night before his arrest.
Human subtlety...will never devise an invention more beautiful, more simple or more direct than does nature, because in her inventions nothing is lacking, and nothing is superfluous.
~ Richter II, The Notebooks of Leonardo Da Vinci, Vol. 2. p. 126 no. 837.
I have been impressed with the urgency of doing. Knowing is not enough; we must apply. Being willing is not enough; we must do.
~ Leonardo da Vinci
First, never underestimate the power of inertia. Second, that power can be harnessed.
~ Richard Thaler & Cass Sunstein. 2008. Nudge: Improving Decisions About Health, Wealth, and Happiness.
October 16, 2017
The Dawn of Social Prediction
"Economists pride themselves on being the most scientific of social scientists. This leads them to reduce all human motives and behavior to quantifiable variables such as utility, welfare and income. But people are not by nature quantitative, and their motives often have no economic basis. Today’s most divisive issues, from fairness and inequality to national identity and culture, don’t have economic solutions."
~ Greg Ip. August 26, 2017. "In Defense of the Dismal Science." Wall Street Journal.
"The American company that promoted the Internet hardest in its early days, Sun Microsystems, conducted research in 1997 into how people read on the Web and concluded simply, 'They don't.' They scan, sampling words and phrases. Why? In part because any one page, on which the fluttering user happens to have lighted momentarily, competes for attention with millions more."
~ James Gleick. 1999. Faster: The Acceleration of Just About Everything. Vintage. p.87.
"In the same way that computers have operating systems at their core — dictating the way a computer works and serving as a foundation upon which all applications are built — everything in life has an operating system (OS). It is at the OS level that we most frequently experience a quantum leap in progress."
~ Bryan Johnson - Founder, Braintree and the OS Fund
"Much of the human experience (knowledge, disease) spreads by proximity, and for any one person the number of elbows in proximity has exploded. In past times, even in the most crowded city, we lived close enough to only a few people to, say, read their journals or track the temperature of their hot tubs. Now, in hordes, they put that information on-line. The multiplication of information pathways leads to positive feedback effects in the nature of frenzies."
~ James Gleick. 1999. Faster: The Acceleration of Just About Everything. Vintage. p.86.
August 26, 2017
The Story-telling Cycle in Markets
Almost 99 percent you realize is just stories in our minds. This is also true of history. Most people, they just get overwhelmed by the religious stories, by the nationalist stories, by the economic stories of the day, and they take these stories to be the reality.
~ Yuval Hariri, Historian and author of the books "Sapiens" and "Homo Deus"
Money is probably the most successful story ever told. It has no objective value. It's not like a banana or a coconut. If you take a dollar bill and look at it, you can't eat it. You can't drink it. You can't wear it. It's absolutely worthless. We think it's worth something because we believe a story. We have these master storytellers of our society, our shamans — they are the bankers and the financiers and the chairperson of the Federal Reserve, and they come to us with this amazing story that, "You see this green piece of paper? We tell you that it is worth one banana."
~ Yuval Hariri
“It’s a motivated decision to say ‘no’ to learning available but unwanted information .... People avoid information if it’s going to make them feel or behave or think in a way they don’t want to”—especially any evidence that could jeopardize their belief in their competence and autonomy or could require taking difficult or prolonged action."
~ Jason Zweig citing Jennifer Howell, a psychologist at Ohio University, in his excellent column in the WSJ
On the first trading day of this year Valentijn van Nieuwenhuijzen’s laptop pops open. He sees right away that something special is going on. The MarketPsych Indices, the system by which NN Investment Partners [formerly ING] measure sentiment in the market, are coming in…words that are associated with stress and gloom course through digital media...
The main strategist of the Dutch asset manager, with € 187 billion under management, taps the brakes: he reduces investments in shares and raises more cash. Later that week the stress spreads: worldwide stock markets went down sharply...
~ Lenneke Arts & Jeroen Groot. February 28, 2016. “Beleggen met big data wordt langzaam gemeengoed.” Financial Daily. (Translated from the Dutch).
June 26, 2017
Switzerland, Neurofinance, and When to Sell in a Bull Market
“When the music stops, in terms of liquidity, things will be complicated. But as long as the music is playing, you’ve got to get up and dance. We’re still dancing."
~ Chuck Price (then CEO of Citigroup) to the Financial Times on July 9, 2007.
"The current level of the CAPE ratio 'would suggest reducing your holdings of stocks, especially for a long-term investor. We can't time the market accurately, but we know that when it's this high, over the long term, it usually doesn't do great.'"
~ Robert Shiller quoted in CNBC, February 24, 2017
"Merchant: In this chaos of opinions, which one is the most prudent?
Shareholder: To go in the direction of the waves and not to fight against the currents."
~ Joseph De La Vega. 1688. "Confusion de las Confusiones." (The first book about the operations of a stock market, the Amsterdam Exchange).
April 02, 2017
Is Trump Playing Crazy? Negotiation, the Game of Chicken, & Dollar Sentiment
To achieve their mating goals, male elephants will sometimes play games of chicken, with one individual essentially giving the impression that he is crazy and has become an irrational player in a game premised on shared rationality and predictability.
~ Professor David Barash, Op-Ed in the NYTimes, 2011
Fittingly, this Year of the Rooster corresponds with a surge in a cock-inspired game in international negotiations: playing chicken.
As a young man I occasionally stood on the bridges across the Rio Grande, practicing Spanish and watching Mexicans commute to work in the United States. They bagged their clothes, paid a peso or two to a man with an inner tube, and were gently pulled across the river under the eye of inert border patrol agents. U.S. customs wasn't apparently concerned enough to intervene. I myself climbed under the fence or waded the river a few times out of convenience or curiosity. Even the Laredo, Texas INS chief's nanny was well-known to commute to work that way. In subsequent years the border has obviously tightened. And now we're hearing about The Wall, whose conception has badly punished the Mexican Peso and local stocks.
As active investors it is our job to predict trends and reactions in global economies and markets. Donald Trump's spontaneity has introduced a degree of unpredictability to our work. His negotiations around trade agreements, immigration policy, tax policy, military arrangements (NATO), and budgetary allocations are all going to be significant to the markets over the next year. Whether one agrees with Trump's proposals or not, it's important to know how he will pursue them, when he will go full force, and when he might back down.
Trump's tactics are familiar - and perhaps predictable - to students of game theory. Today's newsletter looks at this important topic - one we have addressed previously - the psychology of negotiation and playing chicken. Later we look at a turn in U.S. Dollar sentiment around the U.S. election and its aftermath.
Game Theory in the Real World
John Nash is the subject of the Academy Award-winning movie "A Beautiful Mind" and the father of Game Theory involving multiple equilibria in non-cooperative games - exactly what we need to understand the psychology of playing chicken.
In
It's a tactic that works surprisingly well, because male elephants can in fact become temporarily "crazy". One of the most terrifying sights in the animal world is an elephant in a state of must: Huge bulls, oozing a weird, foul-smelling, greenish glop from glands near their eyes, behave with violent abandon, taking risks and defying the basic rules of pachyderm propriety (and also giving rise to the term "rogue elephant"). Facing an elephant in must, other elephants — not to mention people — are well advised to get out of the way.
Dr. Barash explains that by playing chicken - as Donald Trump may be inclined to do as he renegotiates terms of existing trade agreements - low-power players can extract additional concessions due to their hardliner stance. As he puts it, "The trick to winning is for one player to convince the other that under no circumstance will he or she veer off course." Dr. Barash continues, "Another tactic, favored by the strategist Herman Kahn, is to "throw out the steering wheel," to demonstrate that you are locked into a certain path and can't swerve."
January 29, 2017
2016
The Seasons of Social Change, S&P 500 Sentiment Rebound, Perspective-shifting
As Brexit before it and the Italian referendum following it, November's U.S. election represents a social turning point. Some believe the light is fading across Western democracies. Others sense renewed engagement after a long period of public passivity. Both camps see this as a pivotal moment in history.
It's not only democratic engagement that's at a turning point. This time of year, across the northern latitudes, nature is growing quiet. Plants and animals have stored away food for the cold months and months of dormancy. When the snow comes, the quiet will be even deeper. During the darkness of winter, this week's solstice marks a turning point in the season. December's holidays symbolize the renewal of life, light, and growth from the depths of darkness.
After the November U.S. elections a rapid shift in media sentiment strongly correlated with the rally in U.S. stock prices, and this was surprising to many market-watchers, who anticipated a large correction if Donald Trump was elected.
Both around strongly felt events and during the dark of winter, the human mind is susceptible to inertia, becomes trapped into believing narratives (E.g., "Trump/Hillary means the end of the world") or ("maybe the light will never return"), and the mind has difficulty shifting perspective. For the seasonal darkness, societies developed light-themed holidays (Diwali, Hanukah, Christmas, Santa Lucia) to break through inertia during dark days. For individual investors, some top performers use a technique called "reappraisal" to prevent inertia. When predicting market prices, impartial machine learning-based systems are proving to be a valuable tool when the majority of human investors are stuck in a rut.
Today's newsletter examines sentiment shifts around events, the power of machine learning to take advantage of these, and a technique used by top investors to avoid mental inertia.
Did Artificial Intelligence Predict the U.S. Election?
Although the darkness will continue to lengthen into the solstice this week, light will begin its return on the 22nd. We described a similar reversal phenomenon in market prices in our last newsletter. A sell-off into an uncertain event such as an election typically reverses in the subsequent period. Sometimes prices reverse after the event because the sense of dread and foreboding is gone, replaced by relief that the event has passed. Sometimes prices revert upwards because of growing optimism about business conditions. Probably both of those occurred following the U.S. election, juiced onwards by investors who had sold out in advance to reduce their risk exposure, and now jumped back in out of fear of missing out on the post-election rally.
The following image depicts our financial media-derived sentiment data versus the S&P 500 daily prices this year. From a low point in November before the election, sentiment jumped impressively in the hours following Trump's victory.
Such rapid sentiment reversals are difficult for humans to time accurately. We tend to become absorbed into the story around the events. Such event-inflexibility provides an opening for machine-learning-based predictive models.
As we mentioned in our September newsletter, we've developed such a machine learning based model that makes predictions on the S&P 500, and it was extraordinarily accurate not only in the two weeks around Brexit, but also in the week of the U.S. election and the following week. It was long the day before the election, went short the day of the election through the following opening, and was long over the following week. However, the system is not perfect. It had periods of poor performance in October and so far in December this year - please see disclaimers below. It especially shines around major psychology-driven events such as corrections and referenda.
Our machine learning-based model probably performs so well around uncertain events because it can impartially take advantage of the human biases that blind the majority of traders. While these collective biases can be disabling, some top investors have found ways to reduce the impact of such vulnerabilities via a practice of "perspective-shifting."
Perspective-Shifting
In his “Belief in Fallibility" George Soros (1995) explains that to others being wrong is a source of shame. But for him, recognizing his mistakes is a source of pride. Soros explains that realizing that imperfect understanding is the human condition leads to no shame in being wrong, only in failing to correct our mistakes. It requires remarkable courage to consider one's imperfections and mistakes impartially. And evidence suggests that the ability to reappraise one's circumstances is an important tool for reducing common financial biases.
Researchers have found that cognitive reappraisal reduces the impact of loss aversion on decision making (Sokol-Hessner et al, 2009). Reappraisal refers to readjusting one's perspective of a situation along several dimensions. In an experimental condition, researchers randomly asked subjects to think of / review their individual investments in the following contexts: (1) as part of a portfolio, (2) as one of many in a series, (3) as part of a routine job, (4) as expecting that losses are going to happen (“you win some and you lose some”), and (5) as not having direct consequences for their lives. In aggregate these cognitive reappraisals reduced both physiological reactions to losses (measured via galvanic skin response) and subsequent loss-aversion (biased behavior). According to Sokol-Hessner (2009), “‘perspective-taking,’ uniquely reduced both behavioral loss aversion and arousal to losses relative to gains, largely by influencing arousal to losses.”
In summary, Soros is right. To improve ourselves as investors we must learn from our mistakes, and the best perspective to take is one of curiosity and flexibility. We must shift our perspective from one of "Oh no, another bad mistake!" to "Great, another opportunity to learn!"
When approaching losses, thinking about the loss as 1) a routine part of the job of investing, 2) as one of many that one will experience over a typical career, and 3) as not affecting one's physical life or health, will help normalize the loss and improve subsequent decision making.
Houskeeping and Closing
We are approaching the dead of winter in the northern climates. The holidays ahead teach us to maintain hope in the darkness and to celebrate the value of persistence as we carry the light forward into the new year.
Despite the impressive results of machine learning in financial markets, the victory of the machines is not yet at hand. Machine learning thrives in environments where humans do not, such as around psychologically fraught events (elections, volatility, etc...). Where humans are inflexible, machines prosper. Taking a larger view, these periods of inflexibility can teach us about our own weaknesses such as our propensity to make mistakes in markets with fast-moving prices or when we believe fixed narratives about how things should be.
We love to chat with our readers about their experience with psychology in the markets. Please send us feedback on what you'd like to hear more about in this area. Read more about individual psychology in our books "Inside the Investor's Brain" (Wiley, 2007) and "MarketPsych" (Wiley, 2010). Market psychology and sentimental markets are examined in our newest book "Trading on Sentiment: The Power of Minds Over Markets" (Wiley, 2016).
If you represent an institution, please contact us if you'd like to see into the mind of the market using our Thomson Reuters MarketPsych Indices to monitor real-time market psychology and macroeconomic trends for 30 currencies, 50 commodities, 130 countries, 50 equity sectors and indexes, and 9,000 global equities extracted in real-time from millions of social and news media articles daily.
Always seeking new perspectives,
Richard Peterson M.D. and the MarketPsych Team
References
Sokol-Hessner, Peter, Ming Hsu, Nina G Curleya, Mauricio R. Delgado, Colin F. Camerer, and Elizabeth F. Phelps. 2009. “Thinking Like a Trader Selectively Reduces Individuals’ Loss Aversion.” Proceedings of the National Academy of Sciences 106 (13): 5035–5040.
December 18, 2016
How to Invest Through Elections, Product Launches, and Market Uncertainty
While polls show Hillary Clinton comfortably leading over Donald Trump for the U.S. Presidency, Clinton's victory is not guaranteed. Damaging revelations from the hacked DNC emails may still emerge. And perhaps silent Trump supporters are not forthcoming with pollsters, quietly hiding their voting intentions as pro-Brexit voters did.
Events with uncertain outcomes periodically pierce the calm of financial markets. Such events rivet attention and distort prices. We've seen dramatic price moves this year on the back of the Brexit vote. The U.S. Presidential election (and later European referenda) could hold similar surprises.
Such uncertainty about events can generate predictable patterns in financial market prices. Today's newsletter explores the nature of event-related uncertainty, how investors and prices respond to it, and how to best position ourselves around it.
Betting on the Unexpected
Markets are constantly in a state of uncertainty and flux and money is made by discounting the obvious and betting on the unexpected.
~ George Soros
The future is never clear, and you pay a very high price in the stock market for a cheery consensus. Uncertainty is the friend of the
buyer of long-term values.
—Warren Buffett
The fundamental law of investing is the uncertainty of the future.
~ Peter L. Bernstein
October 17, 2016
Mass Manipulation, Trading, and the Technology Polarizing Our Societies
"If we understand the mechanism and motives of the group mind, is it not possible to control and regiment the masses according to our will without their knowing about it? The recent practice of propaganda has proved that it is possible, at least up to a certain point and within certain limits."
~ Bernays, Edward. "Propaganda" (1928).
"This is an age of mass production. In the mass production of materials a broad technique has been developed and applied to their distribution. In this age, too, there must be a technique for the mass distribution of ideas."
~ Edward Bernays, "Manipulating Public Opinion" (1928)
"We are governed, our minds are molded, our tastes formed, our ideas suggested, largely by men we have never heard of. This is a logical result of the way in which our democratic society is organized. Vast numbers of human beings must cooperate in this manner if they are to live together as a smoothly functioning society."
~ Bernays, Edward. "Propaganda" (1928).
"...In almost every act of our daily lives, whether in the sphere of politics or business, in our social conduct or our ethical thinking, we are dominated by the relatively small number of persons...who understand the mental processes and social patterns of the masses. It is they who pull the wires which control the public mind."
~ Bernays, Edward. "Propaganda" (1928).
September 04, 2016
Violence, Media Contagion, and Investing in a Time of Social Insecurity
When I was training as a psychiatrist, I enjoyed taking overnight shifts in psychiatric emergency rooms. In the Psych ER my job was, in part, to ascertain whether patients were at risk of hurting themselves or others. If the odds of violence were high, then the individual was hospitalized. If not, their problems were further managed outside the hospital. At its core, it was an exercise in predicting human behavior - violent behavior.
If a patient was downcast, regretful, or hopeless, we were more concerned about suicide. If angry, bombastic, or contemptuous, homicide was more of a concern. By reading body language, asking the right questions, and contacting their associates, we could fit together a risk profile and take what we hoped was sensible action to keep the patient and the public safe. Since I was in training over a decade ago, mass casualty events have surged to epidemic proportions in the US.
Due to the high frequency of global violent incidents over the past few months, today's newsletter will review the psychological dimensions of such events. In particular, we examine how media reports about violence systematically affect our individual mindsets, create a contagion effect on the mentally unstable, and generate predictable patterns in financial market prices. The end of the newsletter focuses on investment opportunities related to violent media coverage in currencies and global stock markets. (Future newsletters will cover sunnier topics, I promise).
Violence and News Media
Lately the news media is saturated with violent news. I'm not just saying that - the data speaks. Below is a chart of the simple 500-day average of our Thomson Reuters MarketPsych Violence index (Violence TRMI from here on out). The y-axis represents the percentage of references to violent crime or war (as a percentage of all references) in a country, its cities, or its regions. The grey line is the Violence TRMI for the US, the green line is the index for the Eurozone, and the blue line is that of China. The date range is 1999 through the present.
July 30, 2016
How to Predict Brexit - the Human Face of Market Risk
"In fact, it was as a journalist who played around with the facts that Mr. [Boris] Johnson first made his name. He was fired from his first reporting job, at The Times of London, for inventing a quote and attributing it to an Oxford professor (who happened to be is godfather)."
~ "Luck Runs Out for a Leader of 'Brexit" Campaign." New York Times, June 30, 2016.
Boris Johnson is a former Mayor of London, Member of Parliament (MP), and was a leader of the Brexit campaign. He was poised to become the next British Prime Minister until engaging in odd behavior last week.
Johnson started his working life in the media. In 1989 he was assigned to report on the EU from Brussels by the Daily Telegraph where he misrepresented the dysfunction of the EU in order to gain readership, and he enjoyed the public's shock at his stories: “I was just chucking these rocks over the garden wall and listening to this amazing crash from the greenhouse next door over in England,” he told an interviewer. Some of his misrepresentations about the EU became conventional wisdom in the UK, and they may have stoked the euroscepticism behind the Brexit vote (and the subsequent global market declines).
At MarketPsych we research how media information moves investors and market prices. Some information that hits markets is well-defined, such as the impact of the nonfarm payrolls number described in this past newsletter. When information fits an understood model, it is taken in stride, and the markets stay calm. But sometimes events shake our fundamental beliefs about the world, and new frameworks for understanding events are needed. Today’s newsletter examines human risk in markets - the urge to independence - and a unique media-based trading model that correctly predicted the market direction around the Brexit vote.
July 03, 2016
The World's Greatest Stock Picker, AMZN, and Keeping It Real
Manny introduced himself to me as "the world's greatest stock picker." He explained that one key to his success was that he only needed two hours of sleep a night. He pored over details in every significant financial publication and in those quiet morning hours when all others slept, he let information percolate. By the morning he had brilliant new insights into the industries and companies that were poised to outperform over the following months. Some of the world's top fund managers subscribed to his research, he told me.
I asked if his clients knew he was housed in prison, in solitary confinement. He explained that of course they didn't, and he asked that I kindly keep his secret. He distributed his stock research through his secretary, who kept his office open.
In the intervening days I checked out Manny's story. Much was true - he was in fact publishing highly-regarded financial research to large AUM clients from prison.
On the surface his research analysis sounded brilliant - the creative ramblings of an out-of-the-box Wall Street-obsessed thinker. But as we talked in depth it became clear that his thought process was laced with irrational and circumstantial connections. He was often confusing wishful thinking with objective analysis. He was hypomanic, with grandiose claims and excessively optimistic projections.
As a psychiatrist I've worked with many people with grandiose delusions. In each case the client has fixed beliefs that are contrary to reality - beliefs that guide much of their waking actions - beliefs that are entirely untrue. Delusions aren't limited to manic prisoners, in fact we spend most of our days navigating the world based on assumptions, many of which are entirely unfounded. Because the financial markets are imbued with uncertainty, assumptions are more dangerous in that environment. Regardless of the fragility in our collective understanding of markets, there are enormous payoffs for those who can discern reality more accurately.
In fact, academic research on trading models finds that most are delusional. "Most of the empirical research in finance, whether published in academic journals or put into production as an active trading strategy by an investment manager, is likely false." ~ Campbell Harvey and Yan Liu, “Evaluating Trading Strategies,” 2014
This quote is particularly relevant to us at MarketPsych because we are restarting our trading business. We're currently trading a unique media-based machine learning strategy and re-registering as an investment adviser. It has been a long road to find a strategy worth deploying capital into, and based on our prior experience, trading delusions can easily become enshrined in predictive models.
Today's newsletter examines the nature of false beliefs among investors, how beliefs shift (with an Amazon case study), honest investment strategy development, and examines what, if anything, we can do to find the truth about what moves markets.
June 10, 2016
Machine Learning, Big Data, and Finding Alpha in the Noise
In quantitative investing, deep learning could be dismissed as a surefire way to overfit on data. However, as we will describe in more detail below, appropriate set up of deep learning can improve results significantly. In particular, learning algorithms that first identify the market context in which their strategies are deployed (the regime), are better prepared to learn how markets dynamically adapt to information flow. Academic research in finance does not yet use deep learning (interdisciplinary research is often slow in coming), but it does support the value of understanding context. For example, research by Elijah DePalma at Thomson Reuters demonstrates that the performance of common investment strategies differs across market regimes, and these differences may be rooted in the divergent mental states of traders in each context (e.g., optimism in a bull market versus pessimism in a bear market).
Historically, many investors have used the VIX to define market regimes as calm or volatile. As DePalma did in the whitepaper linked to above, sentiment can define market regimes. Our own data product - the Thomson Reuters MarketPsych Indices (TRMI) - was built to address the problem of dimension reduction in media flow, in part to improve regime detection. The TRMI quantify and aggregate the information that is directly meaningful and impactful to traders in the form of granular sentiment indexes like "fear" and "joy" as well as macroeconomic indexes like "earningsForecast" and "fundamentalStrength" suggested by a review of the academic literature.
In the new world of machine-learned strategies, most algorithms use a switching mechanism to change algorithms as regimes shift. Given that deep learning is based on the neural basis of human decision making, it helps to consider how such human decision making changes depending on the context. For example, in the midst of market panic, traders think and behave very differently than in the midst of a gradual bull market. A network that generalizes information like a human mind under stress will behave superiorly during a market panic. However, when markets are quiet, a more complex network architecture can ascertain the nuances of information flow and price behavior. Research supports the use of such regime-dependent approaches in more primitive forms (e.g., switching from value to momentum strategies depending on the VIX level).
With the recent explosion of such machine-readable and granular data sets, deep learning is better able to show its value. To support the surge of interest in applying machine learning to vast financial datasets, a new ecosystem - including data such as the TRMI - has arisen.
May 01, 2016
How Fund Managers Trade on Sentiment
Information sometimes hits market prices hard. The non-farm payrolls number, released the first Friday of each month, has a significant impact on the value of the U.S. Dollar and Treasury bonds.
Elijah DePalma, Senior Quantitative Research Analyst at Thomson Reuters, analyzed the millisecond impact of the nonfarm payrolls (NFP) on the U.S. dollar future contract (DXZ4) on one day—December 5, 2014. A minutely chart of the December dollar index future contract below shows that the price impact is nearly instantaneous with the news release.
SOURCE: Courtesy of Elijah DePalma, Senior Quantitative Research Analyst at Thomson Reuters
Dr. DePalma notes that on December 5, 2014, $5.7 million of USD contracts (DXZ4) were traded within 63 milliseconds of the NFP release, and $29 million was transacted within 100 milliseconds.
Information that is not numerical (as Nonfarm payrolls is) that is conveyed in text is more difficult to measure. MarketPsych’s expertise in text analytics allows us to tackle the non-numerical side of information flow – the concepts that influence and bias investors. Our sentiment-based data feed allows us to deeply understand how information causes herding, and when it doesn’t. This feed is called the Thomson Reuters MarketPsych Indices, and it is consumed by the world’s largest quant funds and banks for trading and risk applications.
April 10, 2016
The Trump Effect, Media Attention, and Stock Price Patterns
There's no such thing as bad publicity.
~ Associated with P.T. Barnum, the 19th century American showman and circus owner
Before the premiere of her first reality TV show - a show called The Simple Life, set on a farm - Paris Hilton was a little-known American socialite. Little-known, that is, until someone released an amateur sex tape of her and her then-boyfriend three weeks before the premiere of the show.
Despite the painful inanity of The Simple Life and the crassness of releasing a sex tape 3 weeks before her show’s premiere, Hilton became a media star and a business success who is now worth around $100 million (per Wikipedia).
There's nobody in the world like me. I think every decade has an iconic blonde, like Marilyn Monroe or Princess Diana and, right now, I'm that icon.
~ Paris Hilton
Any publicity - even the moral outrage over a (probably deliberate) sex tape release - was good publicity for her brand. Hilton’s strategy was later repeated by Kim Kardashian and most recently Donald Trump (minus the tape, so far).
If a strategy of grabbing media attention with morally outrageous acts boosts celebrity brands and sways voting patterns, might media attention to companies also boost stock prices? Today’s newsletter looks at the power of attention to drive stock returns. While celebrities appear to be boosted by publicity - any type of publicity - studying the repeating effects of media attention on stock prices reveals more nuanced but similarly broad patterns over time. But before diving into that, a quick plug for our book, launching this month!
March 06, 2016
Oil Price Patterns and Investor Reactions
Daniel Plainview (played by Daniel Day Lewis): Are you envious? Do you get envious?
Henry Brands: I don't think so. No.
Daniel Plainview: I have a competition in me. I want no one else to succeed. I hate most people.
Henry Brands: That part of me is gone... working and not succeeding- all my failures has left me... I just don't... care.
~ There Will be Blood (2007)
In general, statistical analysis of price patterns demonstrates the existence of two opposite sentiment-based patterns in financial prices: overreaction (e.g., panic bottoms and blow-off tops) and underreaction (e.g., trends). The concepts of underreaction and overreaction refer not only to patterns of prices but also to the collective investor reactions to information that fuel such patterns.
As most of our readers are aware, in partnership with Thomson Reuters MarketPsych derives and distributes the Thomson Reuters MarketPsych Indices (TRMI), representing a real-time quantification of emotional and macroeconomic references in the media to 8,000 companies, 52 stock indexes, 32 currencies, 35 commodities, and 130 countries. When the sentiments extracted from such text are statistically analyzed alongside historical price data, insights emerge into how such information moves crowds of investors and prices themselves. Oil is one of the more sentiment-driven asset, based on our testing.
January 30, 2016
Affluenza, Anger at the Government, and National Renewal
Whether a commitment to lose weight, get organized, or spend less and save more, most New Year’s resolutions have a common theme – they require self-control. Our 2013 and 2014 January newsletters focused on personal transformation. The changes working through the global financial system from 2015 to 2016 are broad-based - e.g., a dramatic decline in energy and commodities prices, a sharp rise in the U.S. dollar, and the increasing cost of credit. Companies, industries, and entire countries are being impacted, but many are still in denial, hoping for a return to the good old days. Formerly-fringe politicians are gaining social support. 2016 promises to be an interesting year, one that will witness the spread of old challenges and the emergence of new ones.
Today's newsletter explores social frustration, affluenza, underreaction in the S&P 500, and more to the point of behavioral economics, science-based evidence on how to change society for the better. As usual, it's a long newsletter, and we recommend skipping ahead to sections of interest.
January 06, 2016
2015
The Psychology of Terrorism and the Price Impact of Mistrust
Fear is the path to the dark side. Fear leads to anger. Anger leads to hate. Hate leads to suffering.
– Yoda
Terrorist attacks and rising homicides fray social trust. The decline is trust is not restricted to one ethnic or religious group. Since 2001 among the 16 people arrested for terrorism in Southern California are Irish, English, Spanish, Arab, and Vietnamese surnames. Obviously, terrorist behavior crosses ethnic and religious lines, so we need to look deeper for explanations. First, we 'll look at the social forces and emotions that fuel terrorist thinking, starting with anger.
When all media anger is added together, we see the following plot of anger expressed in relation to the United States as a whole (superimposed on a chart of the S&P 500). To generate this chart, all specific references to anger in relation to a physical location within the United States were collected and quantified from 1998 to the present. Note that anger as a percentage of overall U.S.-focused chatter is now much higher than 1998 through 2001, and it is also higher than it was in 2007-2008 (the two anger averages plotted are 200-day and 500-day averages, so they are very lagged).
First following the Sep 11 attacks on the World Trade Center, and again after the financial crisis, American anger has risen and remains high. However, the United States has a fairly middle-range level of anger on a global basis, as seen in the below heatmap of anger for the month of November, 2015 (El Salvador, Zimbabwe and Uruguay stand out as particularly high in anger):
In a past newsletter we described the role of anger in driving prices. Across cross-sections of stocks trust has an inverse relationship with future price action (low trust stocks tend to rise). Investors ought to buy the angriest and least trusted stocks. Such stocks' prices are more likely to rise going forward.
December 06, 2015
Becoming a Superforecaster, Gold, and Feedback Effects
Most of the empirical research in finance, whether published in academic journals or put into production as an active trading strategy by an investment manager, is likely false.
~ Campbell Harvey and Yan Liu, “Evaluating Trading Strategies,” 2014.
Having access to more information, more quickly, is fundamental to optimal forecasting. Yet we are overwhelmed with such information. IBM estimates that global data volume doubles every 18 months. For example, as of 2013, over 100 billion business emails were sent daily. If computers could autonomously make sense of this data deluge, then the volume would not be problematic. But computers do not understand meaning, and they need human assistance to develop better forecasts from the data.
For the humans who create forecasting models on this data, making predictions is an exercise fraught with risk. The potential problems range across humans biases to data errors to inappropriate application of statistical tools. Perhaps the most common problem is overfitting - a problem made worse by the availability of increasingly complex statistical tools. MIT professor Andrew Lo summed up the problem of statistical overfitting neatly with this statement, "I’ve often said that, you know, whatever back test you’d like to see, I can certainly produce it for you. If you torture the data long enough, it will basically tell you anything you want."
Given the expertise needed to find predictive meaning in the massive volume of global information, and the predictably poor models and forecasts of experts, Phillip Tetlock set out to identify the traits of what he calls Superforecasters - people who are excellent at synthesizing information and making predictions of the future.
November 01, 2015
When Markets and Minds are Inside Out
All right! We did not die today, I call that an unqualified success.
~Fear, Inside Out (Disney, 2015)
On a long flight somewhere over Asia last week I watched the new Disney-Pixar movie "Inside Out." As usual for a Pixar film, the movie is a brilliant piece of entertainment. What surprised me was that it is also a path-breaking exploration into the nature of the unconscious mind. Perhaps amazingly, this children's movie contains lessons for investors, and those are the topic of today's newsletter.
Inside Out is set in the mind of a girl named Riley from Minnesota. We see the interactions of five manifestations of her emotions — Joy (yellow in the image below), Sadness (blue), Disgust (green), Fear (purple) and Anger (red). The emotions influence Riley's experiences, behavior, and memories via a control console. Joy acts as the dominant emotion to keep Riley in a happy state, but when Riley is 11 years old, her family relocates to San Francisco. Riley has trouble at her new school, and Joy loses control of Riley's experiences. The premise of the movie - that emotions drive most of our behavior - is supported by modern research. Despite this seemingly esoteric topic, the movie is very popular, having grossed over $774.5 million worldwide as of September 27th.
Just as Riley's life was moving along happily until the shock of her move to San Francisco, market prices usually plod along in fairly rational ways - happily on autopilot - until a crisis occurs. While on autopilot, markets are susceptible to underreacting to negative information, giving emotionally-saavy investors an opportunity to act before others understand what is happening. During a crisis, anger or fear dominate investors' collective behavior, creating patterns that investors can identify and take advantage of. Today's newsletter examines how both happily "rational" periods and those of crisis exhibit price patterns. The letter also addresses how investors can maintain optimal emotional function and adapt to changes in the dominant market emotions.
# What Moves Crude?
In "Inside Out" the Train of Thought - is depicted racing through the mind while linking memories, plans, and feelings. The train of thought represents one's conscious experience of the world, and it is the foundation of analytical thought. While emotions always color thought, the use of analytical reasoning about these emotions allows one to gain useful perspective. Using our Thomson Reuters MarketPsych Indices, we can analytically examine the effects of emotions - and loaded topics - on prices. We have begun to identify the predictable aspects of both happily rational and negatively emotional markets.
In 2013 we developed a crude oil forecasting strategy using moving average crossovers (MACDs) of a specific type of sentiment index called PriceDirection. As a quick aside, sentiment is difficult to detect for assets like crude oil, in which media commentary may reflect the sentiment of either producers or consumers, whose feelings are diametrically opposed whether prices rise or fall. As a result, we find that discussions of the price action itself is a more useful sentiment indicator for crude oil. For example, when prices are widely discussed to be rising, momentum tends to set in, and they do subsequently rise. We developed a sentiment-style index called the "PriceDirection" index to capture this phenomenon.
Given the sentence, "Crude prices rose today," the PriceDirection Index would receive a positive score (PriceUp = 1) from that sentence. However, if the text analysis engine analyzes this text string: "News of oversupply drove oil prices lower in late trading," the PriceDirection index would receive a negative score (PriceDown = 1). If both sentences occur in the same hour and no other such commentary is received, then the PriceDirection index would be zero value (PriceDirection = PriceUp - PriceDown = 1 - 1 = 0).
Using both short-term and long-term moving averages of the PriceDirection index, our Chief Data Scientist Aleksander Fafula found that when short term discussion leans towards prices falling, they subsequently tend to fall. When short term conversation about prices rising dominates, they tend to rise. This is a recent image, and depictions of this strategy back to 1998 show significant value. In recent years the overall strategy yields a more than 25% average annualized return with a Sharpe ratio > 1, as described in this prior newsletter.
The PriceDirection index does a good job of predicting price trends when there are no dramatic or rare events affecting the oil markets. Joy and pleasant feelings create a trend that carries forward. Good feelings beget good feelings, and vice versa. However, when a militia threatens to blow up an oil pipeline, we see a sharp rise in emotions such as fear. And as fear comes to dominate perceptions, prices overreact.
The best statistical tool to capture the effects of high emotion levels on price behavior is a decision tree. Decision trees examine the effects of extreme levels of one sentiment versus the subsequent price action in the asset. When the fear TRMI is increasing from week to week (rising emotions), at the most extreme level we see 19 samples with a large jump in weekly fear, and these are followed by an average weekly price increase of 3.2% over the subsequent week for crude oil.
However, fear-based mispricings do not remain in market prices for long. When fear is high for one month, crude oil prices subsequently fall. Using monthly averages, in the 19 months since 1998 with the highest fear (top decile), the following month had an average price decline of -5.4% for crude oil, and prices declined in 100% of subsequent months.
When crude oil fear is neither high nor is changing dramatically, then the crude oil price tends to trend, and the PriceDirection indicator is the best predictor of the price at those times of trending. Overall, using PriceDirection alone is a viable strategy, since rare events are, by definition, rare.
# How Feelings Sway the S&P 500
While crude oil is largely trending on autopilot punctuated by periods of fear, the stock market responds to a diverse range of emotional provocations. All together, the emotional tone of information is summarized as sentiment. As sentiment in the media turns, prices tend to follow.
The following chart of sentiment is similar to that of crude oil, but longer averages were used to improve readability since the chart is a longer duration (back to 1998). This simple strategy returns more than double the S&P 500 by going either long or short based on the prevailing sentiment balance about the S&P 500 (it turned negative this year, contributing to our bearish forecast on the S&P 500 in July 2015). This strategy would have held short through the financial crisis and the dot-com collapse.
Aleksander Fafula found that fear is mildly predictive of next-day reversals in the S&P 500. On a daily basis, short term fear is an overreaction for the S&P 500, as it is on a monthly (and daily) basis for crude oil.
# Managing Your Train of Thought
Crying helps me slow down and obsess over the weight of life's problems.
~ Sadness, Inside Out (Disney)
Crowd behavior (a.k.a. herding) occurs when events exert a strong pull on investors. Investors can use the power of conscious thought to reframe events and replace such compelling feelings, thus avoiding the underreaction or overreaction of the herd.
Personality traits represent ingrained emotional habits, and they color one's thinking about the world. Over 28,000 people have taken our free online financial tests since 2004, and the Investment Personality Test is the most popular test. The personality tests measure the Big 5 personality traits: Extraversion vs Introversion, Agreeableness vs Self-interest, Neuroticism vs Emotional Stability, Openness to New Experiences vs Traditionalism, and Conscientiousness vs Spontanaeity. The Big 5 personality traits are not strongly correlated with overall investing performance (several sub-traits are more interesting), but given our large sample size, we are able see a statistically significant correlation between long-term investing success and two traits: Emotional Stability and Openness to New Experiences (Openness).
If you find yourself low on Emotional Stability, consider whether this is a bad thing. Many people find the full experience of emotions useful, as it helps them to be more sensitive to their environment. At the same time, when emotions become extreme for a long period, poor decision making may result. For investors it is useful to avoid situations that will provoke damaging emotions. Preventive stress management - healthy routines and rhythms to your day - can minimize the likelihood overwhelm.
The second identified performance trait - Openness - is strongly correlated with mental flexibility and adaptability, and it is a trait of top investors such as George Soros (e.g., his Open Society Institute).
As Riley does in the movie "Inside Out," redirecting one's train of thought (cognitive reframing) allows one to dwell on topics or memories that create a productive state of mind. At the same time, as Riley experiences in the movie, one ought not to use cognitive reframing to avoid nagging problems, as they will only grow worse if continually reframed without relieving the emotional core of the issue.
# Housekeeping and Closing
Joy: Come on, group hug! You too, Anger.
Anger: Don't touch me.
~ Inside Out (Disney)
As go the forces that drive Riley's behavior in Inside Out, market prices are either 1) floating along quietly, driven by reasonable expectations while underreacting to new information or 2) dramatic, emotional, and characterized by overreaction. We see predictability in both contexts, but while using very different TRMI indexes. Broad feelings drive markets during #1, while negative emotions like fear are the best predictors during #2.
We love to chat with our readers about their experience with psychology in the markets. Please send us feedback on what you'd like to hear more about in this area.
Please contact us if you'd like to see into the mind of the market using our Thomson Reuters MarketPsych Indices to monitor real-time market psychology and macroeconomic trends for 30 currencies, 50 commodities, 130 countries, 50 equity sectors and indexes, and 8,000 global equities extracted in real-time from millions of social and news media articles daily back to 1998.
Celebrating all of our investing emotions,
The MarketPsych Team
October 03, 2015
The Dynamic of Panic
No passion so effectually robs the mind of all its powers of acting and reasoning as fear.
~Edmund Burke
Humans are predisposed to fearful overreaction. Research on individual propensity to fear and the serotonin transporter gene was featured in a prior newsletter. It is not only static factors like genes that affect risk tolerance. Changes in stress hormone levels - specifically chronic increases in cortisol - also change the risk tolerance of investors.
In a paper in the Proceedings of the National Academy of Sciences, "Cortisol Shifts Financial Risk Preferences," researchers find that humans are more concerned about risk after being subjected to sustained stress: "Specifically, the stress response calibrates risk taking to our circumstances, reducing it in times of prolonged uncertainty, such as a financial crisis. Physiology-induced shifts in risk preferences may thus be an underappreciated cause of market instability."
Chronic increases in stress hormones lead to cognitive shifts such as probability neglect, in which a small probability of a catastrophe becomes overweighted in the decision making process. As fear increases and prices fall, a positive feedback loop occurs in which 1) attention is riveted onto short-term news and information flow and 2) media chatter about the crisis escalates. These two factors amplify each other, raising stress hormones and decreasing investor risk tolerance. A selling climax, in which the price action crescendos into a sharp panic, results. The peak of the panic is a price capitulation in which investors are collectively selling in fear.
September 06, 2015
Innovation, Vision, and Stock Prices
I cannot help fearing that men may reach a point where they look on every new theory as a danger, every innovation as a toilsome trouble, every social advance as a first step toward revolution, and that they may absolutely refuse to move at all.
~ Alexis de Tocqueville (1840)
The past 10-15 years has seen an explosion in innovations: self-driving vehicles (Google), all-electric vehicles (Tesla), inexpensive space launches (SpaceX), the sharing economy (Uber and AirBnB), genetic technologies and cancer immunotherapy, online education (often free), drones, smart phones (Apple, etc...), 3-D printing, robotics, maker spaces, the internet of things, and machine learning - which is itself opening up entire new fields including robotics and translation - let me catch my breath here. Regardless of these amazing innovations over the past decade, some experts believe that these are simply enhancements, not game-changing innovations. They debate whether the economy has reached a plateau of innovation.
Tyler Cowen, a professor of Economics at George Mason University and author of the MarginalRevolution blog, authored "The Great Stagnation: How America Ate All the Low-Hanging Fruit of Modern History, Got Sick, and Will (Eventually) Feel Better," a pamphlet published in 2011. His work argues that the American economy has reached a technological plateau and the factors which drove economic growth for most of America's history are spent. The "low-hanging fruit" from the title include the cultivation of previously untilled land, the spread of transport, refrigeration, electricity, mass communications, and sanitation innovations from 1880-1940, mass education, and potentially cheap fossil fuels and the strength of the American constitution. While those produced extremely large returns, future advances will be much more incremental. In a vivid example, he compares the changes witnessed by his grandmother versus those over his own lifetime, and finds the changes he has witnessed to be incremental rather than world-changing.
Cowen takes a critical and too-narrow view of some recent innovations. He noted on his blog about the hyperloop, a technology to transport people through pneumatic tube-like systems at around 500mph: "I cannot speak to the technical issues ... but I wonder where the real gain is.... You can already fly LA to San Francisco in about an hour. Is saving that time so important, noting that in the best case scenario the hyperloop still takes 35 minutes?"
Cowen's argument neglects that one of the most important aspects of inventiveness and innovation - the DREAM. People - employees, investors, regulators - are motivated by vision. If we deconstruct the vision into its parts - what is feasible with current technology and what is not - we will never try. And even when a new project fails, it often leads to new spin-off technologies and greater wisdom about what works and what doesn't. Steve Jobs understood this, and so does Musk - as he demonstrates when he requires nay-saying employees to explain the materials chemistry and physics that prevent an idea from being manifested in technology. Once the physics makes an idea in the realm of possibility, then the emotional inspiration of the vision is the motivation behind its ultimate achievement.
Ashlee Vance notes that Musk has two posters of Mars outside his office at SpaceX. One is Mars now - red rocks and dust - and one depicts Mars transformed into a viable environment for human life - blue and green and with an atmosphere. That VISION is tangible and emotionally resonant. It is a guiding inspiration for his commercial ventures.
We are on the verge - within the next 30 years - of living in a world of Mars colonies, cancer cures, free online education, travel by hyperloop, a world of self-driving vehicles and drones. Regardless of naysayers, the visions that succeed over the next decades will be those that inspire. Many innovations are possible but have simply not been dreamed of. Those that are brought to life will be those that inspire smart people to dedicate their lives.
August 08, 2015
Risk-on? How fear triggers sell-offs and when to catch the falling knife
Investing is an activity of forecasting the yield over the life of the asset; speculation is the activity of forecasting the psychology of the market.
~ John Maynard Keynes
On the morning of May 6, 2010, mayhem erupted on the streets of Athens, Greece. Later that day, the Flash Crash occurred, sending the U.S. stocks markets down by 9% intraday. A random coincidence? The data suggests not.
Like that May day in 2010, the spectre of default risk raised its head this month. Chinese equities plummeted, Grexit appeared imminent, and even little Puerto Rico contributed a scare. Institutions have a limited power to save us from ourselves, as this excellent article by Jason Zweig recounts, and investors were again reminded that the surges of emotion through markets - especially fear- can drive prices dramatically. A global risk-off movement took hold briefly, and given the disquiet produced by events in China and Europe, it is probable that the volatility is only beginning.
Risk-on behavior is a collective pursuit of financial safety, while risk-off describes the flow of capital to momentum-driven and higher yielding securities. Periods of risk-off are catalyzed by a growing sense of unpredictability, increasingly negative news flow, and rapidly falling prices.
Today's newsletter looks at how a seed of doubt, once germinated, can begin to choke investors with tendrils of fear. We examine a sentiment-based sell signal in China before the recent downturn which is similar to a signal seen before the May 2010 Flash Crash. The question for investors on the sidelines of such panics is when to "catch the falling knife" and buy into the fear. Evidence shows that Warren Buffett is correct to counsel us to "buy on fear," but ONLY when fundamental valuations are also attractive, which we demonstrate through recent research.
July 12, 2015
Greek Tragedy, the Predictive Power of Scandal, and Rewriting an Investing Truth
The question for the markets this month - will Greece default? In the media we hear a morally righteous tale that Greeks should shape up and pay back what they owe. We don't often hear the rationalizations of the Greek side - that other European banks were foolish to lend to a country with such a long history of default and unsustainable deficits. Yet, they were, and here we are.
Austerity makes the economic and psychological damage worse for Greeks, and it not only impairs the ability to repay, but it is also unnecessarily punitive. With a mandate from Greek voters to spurn the austerity program and the reticence of creditors to bend due to fears of moral hazard, the only sensible way forward is default. Greece simply cannot (and should not) pay what it putatively owes. If default happens this month, would all be lost in "Europe's Lehman moment?" Would that shattered trust lead to plummeting stock markets and interest rates and a massive global flight to safety? It has boiled down to a crisis of trust, and for that reason, it presents tremendous opportunity.
In an earlier newsletter we examined how mistrust in leadership is a contrarian predictor of stock prices. In today's newsletter we discuss shattered trust generally. Trust is more resilient than might be expected. And for investors, shattered trust presents tremendous opportunities.
June 14, 2015
Our Age of Speculative Manias & Making Sense of Chinese and Biotech Stocks
Evidence of bubbles has accelerated since the [2007-2009 financial] crisis.
~ Robert Shiller ("Irrational Exuberance," 2015).
The celebrated author and humorist Samuel Clemens (pen name Mark Twain) documented his experiences in the Nevada mining stock bubble, and his writings are one of the earliest (and certainly the most humorous) firsthand accounts of involvement in a speculative mania.
After a brief stint as a Confederate militiaman during the beginning of the U.S. Civil War, Clemens purchased stagecoach passage west, to Nevada, where his brother had been appointed Secretary of the Territory. In Nevada, Clemens began working as a reporter in Virginia City, in one of Nevada’s most productive silver- and gold-mining regions. He enviously watched prospecting parties departing into the wilderness, and he quickly became “smitten with the silver fever.”
Clemens and two friends soon went out in search of silver veins in the mountains. As Clemens tells it, they rapidly discovered and laid claim to a rich vein of silver called the Wide West mine. The night after they established their ownership, they were restless and unable to sleep, visited by fantasies of extravagant wealth: “No one can be so thoughtless as to suppose that we slept, that night. Higbie and I went to bed at midnight, but it was only to lie broad awake and think, dream, scheme.”
Clemens reported that in the excitement and confusion of the days following their discovery, he and his two partners failed to begin mining their claim. Under Nevada state law, a claim could be usurped if not worked within 10 days. As they scrambled, they didn't start working, and they lost their claim to the mine. His dreams of sudden wealth were momentarily set back.
But Clemens had a keen ear for rumors and new opportunities. Some prospectors who found rich ore veins were selling stock in New York City to raise capital for mining operations. In 1863, Clemens accumulated stocks in several such silver mines, sometimes as payment for working as a journalist. In order to lock in his anticipated gains from the stocks, he made a plan to sell his silver shares either when they reached $100,000 in total value or when Nevada voters approved a state constitution (which he thought would erode their long-term value).
In 1863, funded by his substantial (paper) stock wealth, Clemens retired from journalism. He traveled west to San Francisco to live the high life. He watched his silver mine stock price quotes in the newspaper, and he felt rich: “I lived at the best hotel, exhibited my clothes in the most conspicuous places, infested the opera. . . . I had longed to be a butterfly, and I was one at last.”
Yet after Nevada became a state, Clemens continued to hold on to his stocks, contrary to his plan. Suddenly, the gambling mania on silver stocks ended, and without warning, Clemens found himself virtually broke.
"I, the cheerful idiot that had been squandering money like water, and thought myself beyond the reach of misfortune, had not now as much as fifty dollars when I gathered together my various debts and paid them."
Clemens was forced to return to journalism to pay his expenses. He lived on meager pay over the next several years. Even after his great literary and lecture-circuit success in the late nineteenth century, he continued to have difficulty investing wisely. In later life he had very public and large debts, and he was forced to work, often much harder than he wanted, to make ends meet for his family.
Clemens had made a plan to sell his silver stock shares when Nevada became a state. His rapid and large gains stoked a sense of invincibility. Soon he deviated from his stock sales plan, stopped paying attention to the market fundamentals, and found himself virtually broke.
Clemens was by no means the first or last person to succumb to mining stock excitement. The World’s Work, an investment periodical published decades later, in the early 1900s, was beset by letters from investors asking for advice on mining stocks. The magazine’s response to these letters was straightforward: "Emotion plays too large a part in the business of mining stocks. Enthusiasm, lust for gain, gullibility are the real bases of this trading. The sober common sense of the intelligent businessman has no part in such investment." (from Meir Statman)
While the focus of market manias changes - mining, biotech, Chinese stocks, housing, etc… - the outline of a speculative bubbles remains remarkably similar over the centuries. Today's newsletter examines the latest research into speculative bubbles and looks at how we can apply that knowledge, with examples of the recent booms (bubbles?) in Chinese stocks and Biotech. This newsletter is much longer than usual letter, in part because the topic is both complex and important. Skip ahead to the end for the Chinese and Biotech conclusions. A more thorough treatment of bubbles is in my next book, coming out in late 2015.
May 03, 2015
Belief, Rigidity, and the Power of Critical Thinking
“Herbalife: the customers are fictitious, the business opportunity is a scam, the university degree is a fraud.”
~ Bill Ackman
Multilevel marketing preys on a suspension of disbelief. The radio show “This American Life” ran a report on a multilevel marketing venture called “WakeUpNow” (WUN). WUN’s business was very difficult for the reporters to ascertain. It appeared to be cultivating a positive belief that one could convince others to hold the same positive belief (and pay for a monthly subscription while doing so). The radio story about the company reveals the psychology behind the multi-level marketing of belief. The company now appears shuttered.
Herbalife (HLF) is the best-known multi-level marketing company. Bill Ackman, a successful hedge fund manager, took a $1 billion short position in HLF in 2012. He claimed that Herbalife is multi-level marketing scam that preys on the poor and the ignorant. Others joined him. Per The Atlantic magazine in 2014, critics claim “Herbalife is targeting groups who are easily victimized by false promises of riches.” On the other side, Carl Icahn accumulated a 17% stake in Herbalife and claimed it was a legitimate business.
Again according to The Atlantic, “Fundamentally, Herbalife targets our cognitive weaknesses, though which weaknesses is a subject of debate.” HLF is not unlike many other businesses (payday loans, tobacco, etc…) that prey on biases. HLF and WUN are selling positive belief – the belief that one can get rich through effort and chutzpah.
April 05, 2015
Intellectual Imposters, Greece, and Emotional ETFs
As a psychiatrist I’ve worked at the fringes of human experience, and I've come to believe that it’s in those edge cases that we sometimes learn the most about ourselves.
Imagine awakening from a concussion to find that your spouse has been replaced by an imposter. Besides a minor headache you have no other problems - just that one. A stranger is pretending to be your spouse. She looks, talks, and lives exactly like your spouse, but you know - deep down - she’s not your spouse. Of course it doesn’t make sense intellectually that your spouse was replaced by a double, but you absolutely feel that way.
Capgras syndrome is the name for this disorder, and it is characterized by a delusional belief that a loved one has been replaced by an imposter. The feelings of strangeness win out over the intellectual understanding that this person looks and acts identically to your spouse.
For those with Capgras Syndrome, a break in brain circuitry disconnects the facial recognition area from the limbic system. The face of a loved one usually elicits an emotional response - joy, excitement, love. But after a blow to the head (or other neurological issue), this feeling is no longer experienced. Patients see their spouse with their eyes, but they don’t feel anything. In fact, while the skin of a normal person shows evidence of physiological arousal (slight sweating) when the image of a loved one is viewed, the skin conductance of those with Capgras’ Syndrome stays flatlined.
Because Capgras’ sufferers see the exact person (“yes, she looks just like my wife”) but don’t feel recognition (“I know she’s not my wife”), a battle ensues between reason and emotion in the brain. You might expect them to get on with life, living with the intellectual assurance that this person clearly must be their spouse. When everyone around them insists “this is your spouse, not a fake!” – that should hold some weight. But the brain accepts emotional input as superior to visual/intellectual input, and the patient remains doubting.
Because the Capgras’ patient feels no connection with the loved one, the only rational explanation (to the brain) is that she must be an imposter, a double. And the mind tends to attribute sinister motives to the imposter: “What did she do to my real wife?!” which can lead to violent behavior. An elaborate story is fabricated out of a simple disconnect.
But Capgras patients are not always upset by the imposters. One man with Capgras reportedly confessed to his priest that he had repeatedly been unfaithful to his wife – he had been sleeping with his wife’s doubles.
As investors we are often confronted with what we know to be true intellectually versus what we feel emotionally. “I know this market is overvalued on a historical P/E basis, but I feel like it will still go up.” Even as experienced investors who know we should rely on the intellectual assessments, the pull of emotional reasoning - reinforced by the media - is strong. As you can see in our ETF model below, the best investing opportunities come when we trade against both the emotions and the intellect of the media. This week’s newsletter examines the battle of reason and emotion, how that plays out in events such as a potential Greek default, and the cycles of belief that drive ETF prices.
March 01, 2015
Magical Markets, Swiss Franc, and Social Earnings Forecasts
We realise that you will think your own experience while watching magic is unique to you, but we know that, in general, everyone thinks in more or less the same way. Even as it gets harder to apply a technological edge, applying a psychological edge offers us nearly limitless possibilities.
~ David Blaine
In his TED talk "How I held my breath for 17 minutes," magician and endurance performer David Blaine recalls several of his jaw-dropping performances. How magicians misdirect the attention and perceptions of groups has lessons for investors, which we will explain deeper in this newsletter.
One of the wonderful things about the art of magic is that it doesn’t really matter where the trapdoor is. That’s not the secret. The secret is that magicians influence what you think by using your own preconceived ideas of the world around you to amaze you.
~ David Blaine
Gustav Kuhn, a cognitive psychologist and magician, studies how magic tricks work. In one study Kuhn threw a ball into the air. When it came back down he caught it, and he threw it back up in the air, his eyes following it up. He did this twice, and on the third throw he pretended to throw it up while his eyes tracked the invisible arc of its course. Two thirds of the participants reported seeing the ball vanish in mid-air, even though it never left his hand. In a second condition, he pretended to throw the ball in the air, but instead of tracking it with his eyes, he stared at the ball in his hand. In that condition only one-third of the subjects believed he had thrown the ball into the air. Participants misperceived the actual event based on their expectations, as set by the magician.
According to Kuhn, "Even though the ball never left the hand, the reason people saw it leave is because they expected the ball to leave the hand. It's the beliefs about what should happen that override the actual visual input."
As humans we are hardwired to perceive patterns and to develop beliefs that even reality cannot shake. Magicians learn how to set beliefs, misdirect attention, and trick our senses. Some of their tricks parallel the ways in which markets collectively fool investors.
In today’s newsletter we review what we can learn from magicians, the untethering of the Swiss Franc (CHF), this weeks’ tech stock earnings, recent quantitative stock results, and when we should believe our market perceptions versus when we should doubt them. As usual it's a fairly long newsletter today, so feel free to skip ahead to the topics or images of interest.
January 30, 2015
Ruble, Oil, Chinese Stocks, & Evolving with Sentiment Regimes
One thing: you have to walk, and create the way by your walking; you will not find a ready-made path. It is not so cheap, to reach to the ultimate realization of truth. You will have to create the path by walking yourself; the path is not ready-made, lying there and waiting for you. It is just like the sky: the birds fly, but they don't leave any footprints. You cannot follow them; there are no footprints left behind.
~ Osho
It’s been over a decade since MarketPsych set out to decipher how sentiment impacts global markets. We dove into text-analytics in 2004 after we saw research indicating that - even when the overall payoff odds are known by investors, they will often choose investments with lower (or even negative) payoffs due to the words used to describe such investments. Such frenzies of excitement and despair about markets - leading to wealth-destroying investor behavior - are expressed in social and news media, and they seemed to us (and many others) to be correlated with market price patterns. We realized that price and fundamental data wasn't enough. We wanted to understand markets on the level of group behavior - consensus and the information flow that drives it.
After good preliminary results from trading social media sentiment on paper from 2006-2008, we ran the MarketPsy Long-Short Fund LP - the first social media-based hedge fund - from 2008-2010. There were some media reports on our fund with an interview here and an overview here. But while our trading was profitable - our fund beat the S&P 500 by 24% over its lifetime and it beat its benchmark by even more - our ability to keep our tech operations capitalized through the financial crisis was not. Turning away from fund management and into data distribution and research, we were fortunate to enter into a partnership with Thomson Reuters, pairing our unique style of analytics with their world-class project management, data handling expertise, and information flow.
As we look back over a decade of our development, we see exciting changes ahead. We're planning to release important new products in 2015, and every week we're finding novel insights into the role of sentiment and information in driving asset prices.
In today's newsletter we explore how sentiment impacts markets with examples from Russia, Crude Oil, and the Chinese stock market. We then explore how sentiment is itself dynamic. The best investing strategies differ in bull versus bear markets, and this excellent Thomson Reuters white paper reveals a dynamic technique to take advantage of this effect. We then explore how emotionality among investors, versus the analytical discussions of fundamentals, is actually one of the best predictors of stock prices. Goal setting for investors is far different than for people in other fields - we wrap-up with a guide to investment goal-setting for 2015.
January 03, 2015
2014
Leadership Psychology and Investing - Buy the Accused, Sell the Acclaimed
They grumbled and complained of the long voyage, and I reproached them for their lack of spirit, telling them that, for better or worse, they had to complete the enterprise on which the Catholic Sovereigns had sent them. I cheered them on as best I could, telling them of all the honors and rewards they were about to receive. I also told the men that it was useless to complain, for I had started out to find the Indies and would continue until I had accomplished that mission, with the help of Our Lord.
~ Christopher Columbus, Wednesday, 10 October 1492
Christopher Columbus had set out 68 days earlier confident that he could reach Cathay (China), but a series of bad omens had spooked the crew, and by October 10th they were mutinous.
After setting out from Spain, they arrived in the Canary Islands to pick up supplies. There they received word that the Portuguese King had ordered the crew captured and imprisoned. Then after embarking from the Canaries, the rudder of the Pinta broke and they had to return to the islands for repairs. While on Tenerife, the island's volcano began to violently erupt - a new and frightening experience for the Spanish sailors.
They took to the seas again, and as the shores of the last of the Canary Islands faded out of sight, many sailors burst into tears, saying that "they were sailing off—off—off—upon the awful Sea of Darkness and would never see land any more" (Brooks, 1892).
The ships slowed in the Sargasso Sea - where there are few winds or currents - and drifted lazily through beds of seaweed and still waters for a week. The sailors became concerned that they would die, drift-less. Mirages of land raised hope and then dashed the crews' spirits.
Columbus soon realized that his calculations were incorrect - they had not found land where he thought they would. If Columbus returned to Spain without having reached land, he would face disgrace, imprisonment, or even death. He began to doctor the distances traveled in his logs, keeping one log of the true distance and one for his crewmen which showed less distance traveled. The sailors noticed that the magnetic compass no longer oriented itself properly to the North Star. To quiet their fears, Columbus lied and told the crew that the North Star had changed its position.
On October 10, 1492 the anxiety of the crew turned into mutinous fervor. To assuage his sailors' fears, Columbus took a gamble. He promised that if they did not sight land within three days, the ships would turn back to Spain.
Fortunately for Columbus and the sailors, landfall was made on the morning of Oct. 12, 1492. Columbus appealed to duty, cajoled, threatened, practiced deceit, and finally made a last ditch gamble, all to maintain his leadership in the pursuit of his vision.
Like Columbus, executives often face restive investors as they pursue their corporate goals. Sometimes they gamble their leadership on a specific outcome - Columbus' "just three more days" becomes their bet that they can turn around earnings "next quarter" or that a new product launch will be a hit. If they achieve their goal they are acclaimed, but if not their reputation may be destroyed.
The social psychology of how we perceive and respond to leaders has deep roots in the human psyche. Powerful positive social emotions about leadership - such as adoration and acclaim - are not far removed from blame and scapegoating. This month's newsletter examines human sacrifice, unfairly blamed leadership teams and their opposite - superstar CEOs, and predictive analytics of CEO comments and how those affect stock prices.
December 07, 2014
When the Perceived Risk Exceeds the Actual Risk & Catalysts
What we are betting on is that the perceived risk exceeds the actual risk. That’s fundamental to the theory of everything we do.
~Wilbur Ross, describing re-insurance investments after Hurricane Katrina
Hurricane Katrina struck the Gulf Coast of the U.S. in 2005, and it was followed by another powerful hurricane several weeks later—Hurricane Rita. After Katrina the media was saturated with vivid images of submerged residential neighborhoods, people stranded on their rooftops begging for help, and bodies floating in the brown water. Katrina was the most expensive natural disaster in U.S. history with total property damage estimated at $108 billion (2005 USD). At least 1,833 people died in hurricane Katrina and the subsequent floods. Insurers were liable for billions of dollars in damage claims, and they raised their premiums over 50 percent each of the following two years.
There was an increasing perception that category 5 hurricanes would devastate this area of the U.S. more frequently. An influential scientific study published in 2005 indicated an increasing trend in the rate of powerful hurricanes in the Atlantic, and Al Gore’s movie, An Inconvenient Truth, about the catastrophic environmental risks of global warming, was released shortly after the hurricanes struck. The 2005 Atlantic hurricane season appeared to imply that worst-case scenarios were coming to fruition even faster than predicted.
Savvy investors, especially reinsurers, smelled opportunity in the high risk perceptions. Both Warren Buffett’s Berkshire Hathaway and billionaire investor Wilbur Ross poured money into Gulf Coast reinsurance enterprises. In a Wall Street Journal interview, Ross explained such investments by stating, "What we are betting on is that the perceived risk exceeds the actual risk. That’s fundamental to the theory of everything we do." Fear irrationally drives up risk perceptions, and savvy investors locate such opportunities and exploit them.
On this Halloween/Dia De Los Muertos weekend, it's worth considering what we fear as investors. Zombie investments that never live up to their potential (a.k.a. value traps)? Ghosts in the machinery of Wall Street that bankrupt us in milliseconds? There are too many risks to track on Wall Street (and in life). Although we cannot understand or anticipate every risk, we can understand when others are going wrong in their assessments.
This month's newsletter is about risk perception, how high risk perceptions create opportunities for investors, and how to identify catalysts that indicate a reversal. We visit the recent decline in crude oil prices - predicted by sustained high risk perceptions - and we'll look at how risk perceptions predict global stock prices and individual stocks over long periods. To take advantage of excess risk perceptions, investors need the courage to doubt others' fear (captured by Wilbur Ross' quote above) coupled with the clarity and patience to identify the issue that, when resolved, will collapse the energy behind the fear - the catalyst.
November 01, 2014
Ebola, Hong Kong, and Trading on Fear
We go out of our course to make ourselves uncomfortable; the cup of life is not bitter enough to our palate, and we distill superfluous poison to put into it, or conjure up hideous things to frighten ourselves at, which would never exist if we did not make them.
~ Charles Mackay, Extraordinary Popular Delusions and the Madness of Crowds, 1841
The first time I ate chimpanzee was in 1996 in Zaire (now Congo). At the time I was traveling across central Africa by road, but the road became impassable. After a failed attempt to canoe the Congo river (as described in the opening of this prior newsletter), with two other travelers we assembled a raft to proceed downriver Joseph Conrad-style.
While traveling down the river on our raft, we ran out of food. We had left Kisangani in a hurry and had lost some provisions to the river rapids. In order to eat we bought bush meat from local hunters. Unfortunately, we soon ran out of coal for our cooking stove. In order to replenish our cooking coal and food supplies, we decided to make a pit-stop in Bumba on the northern bank of the Congo river. We were floating near the southern bank, but visually the northern bank of the river looked only about a mile away on the morning we decided to cross. But that was an island. In fact it was seven miles across, and after an exhausting and hungry paddle, we arrived at the tail end of the city at sunset.
We tied up our raft near an open-air market, and the first food stall I encountered was surrounded by a boisterous group of men around a metal vat full of bubbling stew. One man placed a bowl of meat stew in my hands and encouraged me to eat up. I was half-starving, they were friendly, and the stew smelled delicious.
It was a wonderful dish - smooth with lightly textured meat and spiced exquisitely. The men were asking me questions in broken, heavily-accented French, and it took me a few minutes to understand - as I scraped the last bits from my bowl - that they were asking me if I'd eaten chimpanzee before.
Chimpanzee is a delicacy in central Africa, said to increase strength and virility. Unfortunately I was also aware that the body fluids of infected chimpanzees were the vector that caused the 1995 outbreak of Ebola virus in Zaire, in that same region.
I put down my empty bowl with a grim expression, concern gripping my mind. I interrogated the men in urgency - "Where did you get this chimpanzee?!" "Was the chimpanzee found dead in the forest or killed while alive!?" "Haven't you heard of Ebola fever?!" The men were baffled why I cared about the pre-morbid state of my dinner, and I was unable to get a satisfactory response.
I developed a fever a few days later, and I was seriously worried that I had contracted Ebola. Perhaps, I thought, my death was imminent.
Having feared I would die from Ebola, I know how easy it is to overreact to the danger of an infectious disease. In fact, as humans we're genetically programmed to overreact.
Such overreactions frequently occur in markets. Our hedge fund strategies used to profit from such outbreaks, as you will see below in the Swine Flu example. Today's newsletter focuses on investors' propensity to overreact to danger, the patterns this phenomenon creates in markets, and how self-controlled investors take advantage of such panics. We also have a section on trading strategies and recent events in Hong Kong, and a section on Apple and the psychology of the iPhone 6 release. This newsletter is the fourth in our series on the success traits of top investors, and this month we focus on the management of fear.
October 05, 2014
Brains, Bubbles, and Arbitraging Global Exuberance
Often, on the other hand, Mr. Market lets his enthusiasm or his fears run away with him, and the value he proposes seems to you a little short of silly.
~ Ben Graham, Chapter II, The Investor and Stock-Market Fluctuations, p. 42
Investing legend Benjamin Graham developed a character - the manic-depressive Mr. Market - to account for the emotional swings that course through financial markets. According to Graham, the erratic moods and behavior of Mr. Market provide opportunities. If investors can wait until Mr. Market is in a pessimistic mood and offers a low sale price, then investors who buy his pessimism can increase the expected value of their investments dramatically. Similarly, investors can sell to Mr. Market for an irrationally high price when he is unduly optimistic.
The chart below, explained in more detail at the end of this newsletter, is the equity curve of a strategy that takes advantage of Mr. Market's mood swings across global stock markets. It is modern evidence that the swings of optimism and pessimism matter, perhaps more than any other driver of market prices.
In our prior newsletters we examined two of the top traits of investors: intuitive expected value calculation and adaptability. In today's newsletter we will begin examining the final trait of the three - emotion management. Because this is a lengthy topic, we are splitting emotion management into three additional newsletters: exuberance, fear, and stress management. Today we focus on irrational exuberance: how it throws our analytical prefrontal cortex offline, how to manage it, and how to trade it across global markets.
September 05, 2014
Rule-Based Trading, Adaptability, and When to Admit You're Wrong
Trading has taught me not to take the conventional wisdom for granted. What money I made in trading is testimony to the fact that the majority is wrong a lot of the time. The vast majority is wrong even more of the time. I’ve learned that markets, which are often just mad crowds, are often irrational; when emotionally overwrought, they’re almost always wrong.
~ Richard Dennis
In the early 1980s Richard Dennis was well-known in trading circles - the Prince of the Pits - for having turned $5,000 into over $100m trading commodities through the 1970s and early 1980s. In 1983 Richard Dennis set out to answer the question - "Can trading be taught?" - fueled by a bet with his commodity-trading partner William Eckhardt. In a two-week session Dennis taught the 21 Turtles (Turtle Traders) - who were hand-selected from over a thousand candidates who responded to a newspaper advertisement. Dennis taught them a trend-following trading system with fairly simple and systematic principles such as defined entry and exit criteria, pyramiding of bet size, limited drawdowns, technical entry and exit rules, etc .... The strategy rule-based, and it required discipline in its execution - not unlike systematic CTA strategies of today.
The Turtle trading rules were hoped to be permanently profitable, but like many hopes at the mercy of the markets, the rules didn't pan out. (Note: There is significant disagreement among the sources I found that described the Turtles' trading results, and I'm not sure on which are correct, so I'll try to present the various perspectives in this newsletter).
The search for consistently profitable rules is a Holy Grail of the markets, but according to an analysis by UCLA professor Avandir Subramanyam and colleagues the profitability (alpha potential) of published market anomalies (alpha-generating strategies) rises and falls in 3 to 5 year cycles based on liquidity. And that liquidity is driven by the whims of investors as they deploy capital into such strategies. This liquidity-driven alpha cycle is characterized by 3-5 years of low liquidity and high performance followed by 3-5 years of high liquidity and under-performance.
After a good run up to 2010, most modern systematic CTA strategies - similar in spirit to the Turtles' rules - have had negative performance over the past three years. And as described below, after a long period of outstanding performance, Dennis' trading rules stopped working consistently. We'll explain the Turtles' results in more detail below, but what is clear is that for investors to survive and thrive in constantly shifting financial markets, they've got to remain flexible and adaptable - able to find the alpha opportunities before others, and be ready to move on when their advantage dries up. But like so much in life, balance is the key - investors need to balance such flexibility against the need to stay disciplined and consistent during a temporary setback. How investors deal with these competing pressures - when to look for new strategies versus stick with an older one - is a theme of this newsletter.
This month's newsletter is the second in a 3-part series examining the traits of top-performing investors. Last month's newsletter explored a trait called intuitive expected value calculation. This month we look at adaptability. Adaptability is characterized by mental flexibility, open-mindedness to new ideas, and the ability to reverse one's outlook abruptly when contrary information is available. The greatest investors in history have this trait in common. Later in the newsletter we look at how a failure of investors to adapt in equity and commodity markets - in response to new information - leads to trends that persist over time, creating an advantage for those who can adapt quickly.
August 06, 2014
Thinking Strategically, the Warrior Gene, and Predicting Volatility
It is better to meet danger than to wait for it. He that is on a lee shore, and foresees a hurricane, stands out to sea and encounters a storm to avoid a shipwreck.
~ Charles Caleb Colton
My wife and I are vacationing on the North Carolina Outer Banks this week, and our vacation home was hit by Hurricane Arthur - a Category 2 hurricane. Before the storm we were forced to make a judgment call - should we evacuate voluntarily or stay through the storm?
As the week progressed, the storm was updated from a Tropical Storm to a Category 1 and then to a Category 2 hurricane, and its path was estimated to pass directly over us. An adjacent island (Hatteras) underwent evacuation.
Most vacationers around us chose to stay in their homes out of convenience, or perhaps inertia. "It should be all right" and "Likely just a few twigs blowing around" were two comments I heard from neighbors. Via internet and television we heard widely varying expectations of damage from "light" to "18 foot surge."
The ability to ascertain risk under ambiguity is an essential skill in life, as it is in investing.
July 06, 2014
Market Monsters, Banana Peels, & a Buy-On-Pessimism S&P500 Strategy
He who fights against monsters should see to it that he does not become a monster in the process. And when you stare persistently into an abyss, the abyss also stares into you.
~ Friedrich Nietzsche
When they were younger, my children were afraid of monsters. Usually the monster lurked in the closet, but sometimes it crouched in a dark corner or under their beds. When seen, it looked like a vampire or maybe a witch or both, but most of the time it remained hidden, waiting to strike at the moment they finally relaxed.
For my children the fear was absolutely gripping - the monster FELT real and they positively KNEW that the monster was coming for them.
So I asked, "what's the worst that could happen?"
"It's going to get me," my youngest told me.
"What does that mean?"
"It's going to eat me, until there's nothing left. Until I'm all gone."
As any parent knows, opening the closet and telling a child, "See there's no monster, nothing is going to eat you" doesn't soothe the fear for long. Just because my youngest couldn't see the monster, he didn't feel less threatened. After one such episode - me rationally explaining that we could not see the monster thus it wasn't real - he told me, "it's waiting to come back after you leave."
It finally dawned on me, this monster couldn't be killed with logic.
June 06, 2014
Manic Markets, Nonlinear Thinking, And Getting Your Head Outside the Box
The happiest thought of my life.
~Albert Einstein describing the moment in 1907 he formulated the general theory of relativity.
Most of us have experienced "Aha!" moments, when ideas and feelings click, and our minds work productively and rapidly. Some people experience such episodes again and again, associated with intense joy, elevated self-confidence, little appreciation of risk, rapid thinking, and decreased need for sleep. For some, this is a biological state called hypomania, a lesser version of clinically significant mania.
As a psychiatrist I had friends who had worked on staff at a mental hospital where a renowned comedian was occasionally hospitalized with mania. My friends said that one of the most enjoyable times at their work was when his friend, another celebrity comedian, visited the psychiatric unit. Those two would rapidly take on characters and engage from their perspectives, pretending to be a stodgy old English woman berating a young German boy for stepping on her flowers, or a cowboy asking a deaf, toothless cook to prepare his breakfast. The staff told me their dialogue was funnier than anything those comedians had produced for the mass media (which was itself award-winning).
I once saw a manic engineer from a large high-tech company. The interaction proceeded like this:
Me: "Hello Mr, X, what brings you into the psychiatric emergency room today?"
Him: "7 719 2 619 4639 3 911."
Me: "Um...I don't understand."
Him: "7 263 7621 199 3 7639."
Me: "Hmmm..."
<Five minutes later, I'm still baffled>
Me: "Are you speaking in prime numbers?"
Him: "YES!"
Me: "OK, let's try English now."
He had decomposed every word in the English language into a prime number. (Later, to further demonstrate how perfect his brain state was, he recited the decimals of pi until, after 30 seconds, I asked him to stop).
Mania is a biochemical phenomenon with predictable psychological and behavioral consequences. Manic people feel a wonderful energy - the senses are alive, fear is entirely extinguished, they have little need to sleep, and their self-confidence is supreme. But it is not only individuals who may take on these characteristics, we also see mild manic (and depressive) behavior among herds of investors.
May 04, 2014
How Will We Know When the Music Stops?
When the music stops, in terms of liquidity, things will be complicated. But as long as the music is playing, you’ve got to get up and dance. We’re still dancing.
~ Chuck Prince, then CEO of Citigroup, in an interview with the Financial Times published July 10, 2007
At a party last weekend, I sat for a chat with a few of the guys. The conversation turned to careers, and one man explained, "I've got a great new job with a startup. I'm based in Denver now, flying all over for meetings. The start-up is 15 people, but we could easily be at 150 in a year." He went on to describe what sounded like a very exciting job, but after 5 minutes I realized it wasn't clear what he did for a living, nor did I understand the startup - neither its name, line of business, nor his role in the company.
The conversation was stimulating, but I began to wonder if this conversation was evidence of a bubble. I was reminded me of the shoeshine boy offering stock tips before the stock market crash of 1929.
As we discussed in this past newsletter, bubbles create - and destroy - immense wealth. And as noted here, it is vitally important not to lose the wealth we gain during a bubble. As a result, spotting bubbles and timing their demise is a key discipline to growing and maintaining wealth.
Despite the negative returns in bank prop trading and hedge funds this year, there is finally a party on Wall Street. It took several years post-crisis for stock day-traders (i.e. the investing equivalents of underage drinkers) to emerge. But here they are, enjoying massive volatility (mostly positive) in electric vehicles, biotechs, 3-D printing, and other high-flying stock groups.
Today's newsletter addresses the question - "Are we in an equities bubble?", and if so when should we exit. It goes on to offer a risk management checklist to keep you grounded when your investments are performing well.
April 06, 2014
On the Street in Ukraine, Cartels Falling, and Finding Your Edge on a Cleaner Wall Street
For the past four years two Ukrainian citizens, Eugene Smolanka and Alexey Karakulov, have been MarketPsych software engineers. Both gentlemen are precise, driven, and have unparalleled expertise.
On our company conference call on Wednesday February 26th, Eugene noted that big changes were underway in Kiev, where he lives. Public transportation had been shut down and rivers of protesters were flowing into Maidan Square, a short walk from his apartment. That same day Alexey checked in with us well after midnight. He explained that he had been performing civic duties. Clad in a helmet and wielding a club, he had been protecting protestors marching in his eastern Ukrainian city (Kharkiv). Ukraine's President Viktor F. Yanukovych was voted out by parliament that night.
Throughout the Ukrainian unrest we've been checking the world maps on our website to gauge the intensity of the strife. According to our data, the Ukrainian government was clearly the most unstable in the world the week before the overthrow of President Yanukovych.
The global map above shows the intensity of Government Instability - one of the Thomson Reuters MarketPsych Indices - highlighted in red.
Alexey noted that the emotional fuel for the protests wasn't anger at any one person or political party. The protests were ultimately against unfairness - business cartels, political paralysis, and police corruption - and were stoked by a feeling that the people, all together, could make organic and lasting change. Alexey summed up the mood of the uprising as, "No more unfairness."
After the ouster of Yanukovych, the police stepped back. Per Eugene, "What amazed me a lot is how citizen themselves gathered to stop violence in Kyiv, because police was on side of the titushki [hired thugs]." Eugene noted, "in every district of Kyiv people organized to patrol city and keep peace."
Yanukovych's lavish house was entered by protestors, and Ukrainians were shocked to see video images of his personal zoo, greenhouses, golf course, and the wooden ship that served as his private restaurant. They could see how lavishly the President had been living, presumably at the cost of the country's development.
Now Crimea is poised to secede and join Russia. And given the installation of a new set of oligarchs (from the Ukrainian opposition), whether Ukraine's popular uprising will be sustained is an open question.
On a global scale, the Ukrainian events are evidence of an accelerating paradigm shift. Cartels, corrupt and rigid political systems, and ossified economic regulations are rapidly giving way to open, honest, and free association of people, ideas, and business -- but not without a fight. As investors, we must understand where this trend is heading next so we will not be left behind. Today's newsletter examines how investors can thrive as cartels fall, with an emphasis on finding your edge in increasingly open and efficient markets.
March 06, 2014
Why Not Losing is More Important Than Winning and the Emerging Markets Meltdown
You might never fail on the scale I did,
but some failure in life is inevitable.
It is impossible to live without failing at something,
unless you live so cautiously that you might as well
not have lived at all – in which case, you fail by default.
~ J.K. Rowling
December's newsletter discussed exponential gains in investing, but truth be told, how we handle losing in life and the markets is more important to our long term prosperity than how we approach winning. This newsletter examines at the other half of the investing equation - minimizing your downside.
For most investors these two words - risk management - are a prelude to drooping eyelids and a suddenly urgent need to check one's phone. Yet risk management is ESSENTIAL to good investing. And risk management is all about minimizing the downside. As Warren Buffet - not an investing slouch - noted: Rule No.1: Never lose money. Rule No.2: Never forget rule No.1. And the best way to not lose money is to identify and prepare for potential losses in advance.
February 02, 2014
2013
A Big Year, Goal-setting, and a Vision of 2014
If you don't know where you are going, you'll end up someplace else.
Yogi Berra
The first time our canoe capsized in the Congo river, I immediately called out for my traveling companion. He was a poor swimmer, and we were in the milky brown rapids just above Kisangani (formerly Stanleyville).
The day before we hadn't prepared well for the canoe trip. We had needed to escape town in a hurry as the police shakedowns - common in Africa at that time, 1996 - were intensifying. My buddy elected to buy our dugout canoe himself while I changed money. We might have been OK through the rapids had my companion 1) had canoeing experience (I should have asked), 2) not been stoned when he bought the canoe (sadly he was always stoned, so I'm not sure if it would have made a difference), and 3) bought a larger canoe.
The morning of our launch we tried to escape police detection by arising early and fleeing our hotel at 4am. We loaded our petite canoe on the bank of the Congo at 5am, and set out into the Congo River above the town.
Kisangani is built just below the famous rapids that block further navigation of large boats from Kinshasa. As we watched the lights of the still-sleeping city come into view around a bend, the roaring sound that I thought was insects in the night continued to grow louder. I recalled with dread a few lines from Heart of Darkness: "There were rapids in the river here, and the sound of rushing water drowned out everything else." We tried to turn towards shore, but it was too late. We floated into the rapids, ascended up the crest of a massive surge of whitewater, and slid down into a minor whirlpool, swamping the canoe.
We were lucky after capsizing. I'm a good swimmer, and my buddy managed to haul himself onto our sack of money. (Due to hyperinflation, Zaire's largest bill was worth about $0.20, so we had piles of money stored in a waterproofed bag that floated high and dry. That bag was also a favorite target of the police).
Some events in life make you swear to never repeat them. For me, crossing Zaire in 1996 was one. After surviving that trip, I tried to understand what I could have done differently. I started learning more about political risk, I gained a greater understanding of failed economies, and I looked to better manage the risk of those who make decisions around me. In hindsight, we should have prepared better. And at its core it was a learning experience, which is what all worthy goals are, as we'll discuss later in the newsletter.
Navigating the financial markets is like canoeing across a river. Markets experience periods of calm. Investing in calm markets requires little skill or practice or even awareness of what you're doing. Markets also experience periods of crisis just as there are rapids in rivers. We cannot control the river, and so we must prepare. Within our control is how we learn about and train for contingencies, how we maintain mental flexibility, and how we adapt to unforeseen circumstances. But the chaos of the water itself is completely out of our control.
In 2013 the average hedge fund returned just over 8% according to Hedge Fund Research, while the U.S. equity market (S&P 500) returned over 26% and the Nasdaq over 38%. The stated expected return of most hedge funds is 10 to 20% annually, so with returns of 8% in an extremely positive year for equities, they are sorely lagging expectations. Why?
Every year at this time professional investors set goals and prepare for the new year, and every year they tend to make the same two mistakes. Their recent experiences color their risk forecasts, and they do not prepare appropriate goals. This month's newsletter examines how to set proper goals for 2014, looks at last year's U.S. equities rally, China, and lays out a vision for the year ahead. As you'll see (and hopefully experience) in the next sections, setting effective investment goals involves maintaining a big picture perspective, relying on internal goals, staying mentally flexible, and most importantly, creating a vision.
December 30, 2013
Exponential Wealth Creation and Investor Self-Sabotage
Optionality is the property of asymmetric upside (preferably unlimited) with correspondingly limited downside (preferably tiny).
~ Nassim Taleb, Antifragile
If you 'have optionality’, you don't have much need for what is commonly called intelligence, knowledge, insight, skills, and these complicated things that take place in our brain cells. For you don't have to be right that often. All you need is the wisdom to not do unintelligent things to hurt yourself (some acts of omission) and recognize favorable outcomes when they occur.
~ Nassim Taleb, Antifragile
Most investors have a story of "the one that got away" - a good investments that would have made them a fortune, but alas... Such investments usually possesed the property of optionality, so that the missed return is often several times the initial investment. Like most, I've had a few big misses. For one the President of Dell Computer taught an undergraduate class of mine in Austin, Texas in 1992 and repeatedly told me, "if you want to get rich, buy Dell stock and hold on," but alas the stock's P/E ratio was too high for my valuation models and I was a dogmatic value investor, and alas ... (Dell stock was up 100x over the next 6 years). Even worse, I initially dismissed the internet. I had dinner with my college electrical engineering professors who explained the internet would change everything (this was 1994). At the time I reflected on the painfully slow Mosaic browser and the limited availability of web content, and I vocally disagreed (the memory of my limited vision still hurts). I remember these events because the professors outlined their visions with such wonder. The professors were truly in awe of the world's upcoming transformations.
I learned a few things from these missed opportunities. Each opportunity had enormous - life changing - upside and limited downside. To take advantage of such optionality, we've got to first look for this property. We need the long-term vision to see over the horizon at the exponential changes coming our way. Moreover, we need both the aggressiveness to act on our vision and the prudence to avoid sabotaging ourselves as we pursue it.
Optionality is on my mind lately as Bitcoin and alternative cryptocurrencies (Litecoin, Feathercoin, etc...) continue their ascendance. We described the cryptocurrency bubble in March in this newsletter. In that newsletter you can see a chart of bitcoin as it was about to hit $100 per bitcoin. Today bitcoin is over $1,000 per bitcoin, driven in part by explicit acceptance of virtual currencies by the SEC and Federal Reserve and new Chinese exchanges catering to tech-savvy Chinese moving their savings offshore. Many courageous investors have gained paper (and real) wealth by speculating on cryptocurrencies early, and so today I thought it pertinent to look at the fundamental drivers of wealth accumulation, which is, after all, why we invest.
Today's newsletter explores the creation of massive wealth and the mental biases that sabotage its pursuit.
December 05, 2013
Intuition, AAPL, and Tuning in to the Market's Rhythm
LATEST PRESS
Top Financial Institutions are Regaining Trust News and Social Media Analytics Show. Quartz.
Trader's Tap Twitter for Top Stock Tweets. Oct 11, 2013. James Macintosh. Financial Times.
Curtain Could Fall on Social Media ETF Party" Oct 8, 2013. Trang Ho. Investor's Business Daily.
UPCOMING EVENTS
See us in San Francisco on November 9, 2013 and Orlando November 17, 2013.
INTUITIVE INVESTING
What is it the good managers have? It's a kind of locked in concentration, an intuition, a feel, nothing that can be schooled. The first thing you have to know is yourself.
~ Edward Johnson, Founder of Fidelity Investments
In the quote above Johnson was not entirely correct about one thing. Bad investment managers do not have "locked in concentration, an intuition, a feel" due to the fact that they cannot be learned in school (e.g., in business classes, trainings, etc.). They can be learned, but the problem is that these characteristics have not been properly taught. Although self-knowledge cannot be memorized from a book, skills for developing self-knowledge can be learned.
The goal of today's newsletter is shed some light on the nature of investing intuition.
Feel for the market, trader's intuition, gut instinct, and acting from the zone refer to those times when our investing flows naturally, as if in tune with the market's rhythm. Our thinking is clear. Our reactions to market events are correct. Trading decisions are seamless.
While I'm an empiricist by temperament, intuition is notoriously difficult to study in the context of decision making. We often know when we have an intuitive insight, but when we try to define it specifically, it's an evasive concept. The circularity of intuitive feelings is parodied in this Dilbert comic strip and this one.
Famous intuitives include George Soros and Jack Welch (his 2000 book was titled: "Jack: Straight from the Gut"). Infamous intuitives include Alan Greenspan, who asserted in his 2007 book The Age of Turbulence, "To this day the bathtub is where I get many of my best ideas." Intuitive insights often arrive when we are relaxed, thinking about something completely different from the markets, or perhaps nothing at all. It is in these quiet moments that the collected inputs of the past days and weeks coalesce in our brains, patterns and relationships are recognized, and insights bubble up into our awareness.
November 02, 2013
Buying Default Fears: How Government Paralysis Creates an Opportunity
Safe Haven?
It seems like only yesterday that we analyzed the behavior of prison bullies and the parallels with political brinksmanship in this newsletter.
Today we'll explore the psychology of self-righteous anger and paranoia as it relates to the current political impasse in Washington D.C. – first the government shutdown, and then October 17th debt ceiling and potential default.
The U.S. government political paralysis negatively impacted global investor psychology, driving a flight-to-quality out of U.S. equities last week into the Euro, Yen, Canadian Dollar, and other safe-havens. As we've discussed in prior reports, the game theory behind such brinksmanship requires this game of chicken to end badly, despite the short-term negative outcomes for the economy and political system.
Yet the price movements are not entirely what they were expected to be. The U.S. government shut down last night, and then gold fell more than 2% and U.S. stocks rallied today. What's happening? This is one of the most classic and enduring patterns in financial markets, which will delve into in this newsletter, called "Buy on the Rumor and Sell on the News."
September 30, 2013
The Predictability of Oil Prices, Fear and War
We're All Short Oil
"Oil is like a wild animal. Whoever captures it has it."
~John Paul Getty
I grew up in West Texas, near where wildcatters such as George Bush senior made their fortunes. Oil pumpjacks, rusty and retired after the oil price collapse in the early 1980s, sat idle across the scrubland near my house. For high school kids, those pumpjacks were popular props for spending weekend evenings. There was nothing more pleasant than laying on the cold steel of a pumpjack under the stars, chatting with friends, a bonfire burning far below us.
Being adolescent and thus maintaining our right to poor judgment, it hadn't occurred to us that lighting bonfires on top of decrepit oil pumpjacks could lead to a lethal explosion. It took an incredulous county sheriff to point that out to us one night.
Those disused pumpjacks are pumping again. The bust in oil prices that left such pumpjacks quiet and rusting in the Texas scrub has ended. Sometimes oil price boom/bust cycles are driven by supply and demand imbalances (as in the early 1980s); and other times they are driven by fear and war.
Fear has the opposite relation with oil prices as equity prices. When fear is high in stocks, it is generally a good time to buy. With oil the opposite relationship holds.
Most investors know they are implicitly long the stock market, but sometimes we are long or short assets in hidden ways, making diversification difficult. Just as it took a financial crisis for me to realize that my career is long the financial industry (regardless of how much I was short equities), it often takes a price shock for consumers to realize that - by virtue of their daily heating, transportation, and consumption needs - they are implicitly short oil. Unless your career is in the energy industry, you have a substantial lifetime short position on crude oil.
Fear about oil supply shocks and their reverberations through politics and the economy creates one of the most predictable patterns our analysts have seen in any commodity. This month's newsletter examines three variables we've identified that drive oil prices - Fear, Conflict, and Violence. The letter goes on to give an outlook for oil prices based on the Syrian conflict. But before we jump into an analysis of how fear and conflict drive the oil price, we've got some interesting news.
At MarketPsych Data we've been gradually utilizing our data to augment the academic research on sentiment driving asset prices. We also find that sentiment can be integrated into charts, to improve technical and fundamental analysis. Our new 16-slide presentation demonstrates how sentiment data can broadly be used to improve trading and investment returns with academic references.
Now that our Thomson Reuters MarketPsych Indices (TRMI) are being studied by academic researchers, we're seeing fascinating results start to roll in. Here's a video by researchers at the Stevens Institute of Technology and Thomson Reuters R&D discussing how our data is helping to solve the "Forward Premium Puzzle" in currencies, answering the question, "why does the carry trade work?": http://vimeo.com/72430790.
August 31, 2013
Why Wall Street Needs a Fecal Transplant: Russia, Insider Trading, and Your Microbiome
What Good Are Parasites?
Greek historian Herodotus reported that the ancient
Egyptians used enemas three days per month along the
lunar cycle because they are 'convinced that all the
diseases incident to man have their origin in the food
that he takes'.
~ Gaisford. 1846. The nine books of the history of Herodotus. (Cited in Intestinal microbiota, probiotics and mental health.)
The first time I visited Russia was in 1992, and the U.S.S.R. had recently broken apart. I overstayed my visa - I had entered on a visa for the Soviet Union, but it no longer existed - so I was able to wander around Russia and Central Asia for a few weeks enjoying the fascinating disorganization of the political transition.
Toward the end of my travels in Russia I encountered an old woman sitting on the sidewalk who was selling a single basket of dirty strawberries. That's all she had - one basket of red, dirty, strawberries sitting in the middle of an otherwise empty tablecloth. I hadn't seen any fresh fruit or vegetables up to that point - these were still the years when all fruit and vegetable production went into State-owned factories and emerged peach-colored and flavorless in brown jars. (All jarred fruit in the USSR - regardless of whether it entered the factory as a strawberry, pear, or mango - emerged from the cans peach-colored and flavorless). So craving fresh fruit, I quickly bought the dirt-covered strawberries, scraped off a few chunks of dirt, and popped one into my mouth.
The strawberry seller said something urgently to me in Russian, but I didn't understand. I smiled at her. I proceeded to gulp down another strawberry. At this point several of the vendors around her were also talking to me rapidly. I smiled bigger - my default traveler strategy when not comprehending what was happening - and ate a few more strawberries. They looked at me with a mixture of astonishment, mirth, and concern. I was accustomed to being watched with astonishment and mirth, but their concern was unsettling. Nonetheless, I had no idea what they were trying to tell me, so I left with a spasiba! and a wave. As I walked away the vendors tried to smile at me, but I thought I could see their smiles distorted by underlying grimaces. They were trying to tell me something, I realized. Something was wrong, but what?
The next day I felt a sharp pain in my abdomen, and I involuntarily doubled-over while walking. The stabbing pain went away for a few minutes, but then three stabs of pain returned. Within an hour I was hosting a knife-fight in my gut. By the end of the day each wave of pain led to an explosive event. And as I soon discovered, I had eaten a strawberry covered in human feces.
But more on that later.
This month's newsletter is inspired by a paradigm shift underway in modern medicine. Research into the complex relationship between our diet, our gut microbiome, and our mental and physical health is redefining how we understand human biology. This new understanding has profound implications for how theorists model financial markets and investor behavior.
This newsletter first examines the science of the microbiome-gut-brain axis. We then go on to explore implications of this new understanding for how we think about markets, regulations, and insider trading. In the Researcher's Corner we example an often neglected side of the feedback loop between market prices and investor behavior. We then discuss what you can do to optimize your own health based on the latest science. And finally we review recent psychology-based investment ideas.
August 03, 2013
Youth Rising, Turkish Turmoil, and The Psychology Behind Price Trends
Youth on the Rise
"The very basic core of a man's living spirit is his passion for adventure. The joy of life comes from our encounters with new experiences and hence there is no greater joy than to have an endlessly changing horizon, for each day to have a new and different sun."
~ Christopher McCandless, subject of Jon Krakauer's documentary novel and dramatized film "Into the Wild."
Christopher McCandless was intelligent and accomplished, yet after graduating from Emory University in 1990 he abandoned his material possessions. McCandless adventured in the wild areas of the western United States for two years, and like a modern-day Thoreau, he journaled his thoughts on life, nature, and love. In 1992 he hitch-hiked to Alaska, where he spent a summer living off the land in a remote wilderness. It was in that place he died. His writings often explore a return to simplicity, and for that he remains an inspiration to those disillusioned with the rat-race.
McCandless adopted a free-spirited, non-materialistic existence during a time of economic malaise in the United States - the early 1990s recession. The S&L crisis had exposed a deep vein of banking mismanagement. College graduates had difficulty finding employment, with Physics PhD's working as baristas to make ends meet. Some disillusioned youth hit the road like McCandless, wandering off-the-grid.
Youth in emerging markets such as Turkey and Brazil are disillusioned in a fashion similar to what American youth experienced in the early 1990's. Street protests and capitalist-backlash are a symptom of such economic malaise.
As our S&P 500 Bubbleometer showed in our last monthly newsletter, the U.S. market was vulnerable to a catalyst that would trigger a downside reversal. Bernanke's comment was the trigger. Our weekly commentary reported that the sell-off was likely to extend, as the flames were blown from market to market ... and they did, hitting Brazil especially hard. Now we're nearing a short-term turning point.
The key to MarketPsych's unique research is an empirical understanding of how information and emotion affect investor behavior. To understand social unrest, it helps to peer inside a disappointed brain. In today's letter we examine how disappointment is generated on a brain level to explain the psychology of the protest movements in Brazil and Turkey. Then we move on to discussing the nature of bubble formation and trends. Finally, we examine how understanding the psychology of under-reaction and trend-formation can make you a better investor.
We are very excited to launch our new website! We encourage you to explore the site to make the most of our research and tools. On the basic level, our top lists identify hot spots for fear and optimism across equities and markets. Dashboards allow rapid comparisons and analysis of sentiment and macroeconomic themes across assets. For professionals, our charting tools allow users to investigate how sentiment and macroeconomic themes affect prices, and help users avoid over-crowded investments (saavy investors avoid the consensus). Our researcher platform offers research white papers, weekly updating U.S. equity, currency, and country investment strategies, and a daily-updating 12 country PMI model to help traders see global economic activity in near real-time. We are continually adding new content. Please reach out with any suggestions that would make the site more useful for you.
June 29, 2013
When Will the Rally End? S&P Euphoria, French Frailty, and the Gambler's Fallacy
Betting Against the Trend
"On August 18, 1913, at the casino in Monte Carlo, black came up a record twenty-six times in succession [in roulette]. ... [There] was a near-panicky rush to bet on red, beginning about the time black had come up a phenomenal fifteen times. In application of the maturity [of chances] doctrine, players doubled and tripled their stakes, this doctrine leading them to believe after black came up the twentieth time that there was not a chance in a million of another repeat. In the end the unusual run enriched the Casino by some millions of francs."
~ From FallacyFiles.com: Darrell Huff & Irving Geis, How to Take a Chance (1959), pp. 28-29.
A precious metals bullion dealer called me last year inquiring if I'd do an interview on his radio show about the psychology of precious metals prices. He explained that his customers are irrationally fearful of buying, and he was hoping I would soothe his listeners using something out of my psychological "bag o' tricks." (Note: before you email me that this is unethical, yes, I'm aware of that, and I don't do it).
The broker said he was extremely bullish on metals prices. When I asked why he was bullish, he explained, "Silver is at three-and-a-half year lows, it can't go any lower..." So I asked, "Why not?" and he barraged me with aggressive and profoundly confident assertions: "The U.S. Mint ran out! The demand is far greater than the ETF is holding - they own futures! Silver is an industrial metal and is not recyclable! Retail demand in India and China is just beginning!"
This was the same biased reasoning about silver (and gold) that I heard from others in 2011. When such projective statements are asserted aggressively and without self-consciousness, it is a sign the bubble hasn't fully deflated.
The broker expressed a number of cognitive biases in his thinking about precious metals. I'll discuss one here - the Gambler's Fallacy - since it is being uttered by true believers in many quarters currently: the U.S. stock market ("can't go any higher"), precious metals and Brazil ("can't go lower"), and the Euro ("can't break up") to name a few.
The Gambler's Fallacy is a bias in which investors underestimate the ability of a streak to continue, and as a result, they begin to forecast its reversal based on the length of price action in one direction alone. The reasoning of those embroiled in the Gambler's Fallacy sounds like, "It can't keep on going like this." It is a deeply ingrained bias, and as seen in the opening quote, enriches casinos in Roulette and other games such as Blackjack: "my losing has to turn around!"
On the market-level, the Gambler's Fallacy may influence group behavior, as evidenced by price action around series of events, such as earnings surprises. The authors of this experimental study show how prevalent the Gambler's Fallacy is, and they differentiate it from the "Hot Hand Fallacy" in which streaks are expected to continue due to a talented human with a lucky "hot hand."
Today's newsletter examines how the Gambler's Fallacy permeates reasoning about current market activity in the U.S. stock market, Brazil, and the Euro.
Join us for a free webinar on Friday May 24th at 8am EST on Sentiment-Based Global Investing: "Inside the Global Brain: How sentiment trends in news and social media influence global equity and currency values." Register here.
May 19, 2013
Did We Contribute to the #HackCrash? Light-speed Information and the Psychology of the Herd
Fake Tweet and Tremor in Markets
"Rothschild coaches careered down highways; Rothschild boats set sail across the Channel; Rothschild messengers were swift shadows along the streets. They carried cash, securities, letters and news. Above all, news---latest, exclusive news to be vigorously processed at stock market and commodity bourse."
~ Frederic Morton (1962), "The Rothschilds: Portrait of a Dynasty"
There are many stories and legends surrounding the Rothschild family, and probably most are untrue. It is widely acknowledged that Nathan Rothschild was the first Londoner to receive the news that Wellington had defeated Napoleon at the Battle of Waterloo in 1815. Rothschild received this information through his network of fast carrier pigeons, which one of his agents released from Waterloo when the battle was known to be won.
News of the outcome of the Battle of Waterloo was breathlessly awaited in the British financial markets. As legend has it, when Nathan Rothschild entered into the Exchange the day of the victory, he was already known as a master of speedy information. As such he took up his usual trading position and began quietly selling British Consols (bonds) into the market. Traders quickly identified his selling, spurring a panic. It was assumed by traders that Rothschild had heard Wellington was defeated. Then, legend has it, in the midst of the panic Rothschild began stealthily buying Consols. He purchased a sizable position before traders realized they had been fooled and drove prices higher. Rothschild increased his profit from the victory dramatically by engineering a short-term panic and then buying into it.
This legend serves to illustrate that there are two primary ways to profit as an investor: 1) Get important information fastest, and 2) Know how the herd will react. If you know what the investing herd believes, fears, and expects, then you know how it is likely to react to new information and events.
Back to point 1). Getting relevant information fastest has always been the most straightforward key to successful investing. And the hunt for the fastest information has spawned the term "Algo Wars" to describe the arms race among HFTs for faster servers, co-location, re-engineered routers, and other accoutrements of light-speed trading. The time advantage HFTs use is incredibly narrow. For example, the May 1, 2013 WSJ profiles how HFTs use a 1-10 millisecond advantage to rapidly detect order-flow and front-run larger orders.
At MarketPsych Data our clients include the largest hedge funds in the world, and we can't say exactly how our clients are trading off our sentiment feed. The TRMI are published minutely as a 1440 minute (24-hour) rolling average. And several of our indices do show evidence of the #HackCrash event as you can see below.
To be clear about the title question, I doubt the minutely Thomson Reuters MarketPsych Indices (TRMI) data feed contributed to Tuesday April 23rd's HackCrash in which the S&P 500 plunged 1% and then recovered all within the span of 4 minutes. But I can't be sure. The TRMI both show fast evidence of the event and also give an excellent read on the beliefs and expectations of the investing herd.
Today's newsletter looks at the role of information speed and herd impact. We'll first take a look at a famous failure related to United Airlines, then we'll visit how our systems dealt with the hacked Associated Press tweet and its aftermath, and finally we review a few investment ideas for the week.
April 30, 2013
Gold Psychology, Collapsing Bubble or Short-Term Hiccup?
What Gold Drives Us To...
Man: "You talk as though you struck it rich sometime or other, Pop. How about it? Then what are you doin' in here, a down-and-outer?"
Howard: "That's gold, that's what it makes of us. Never knew a prospector yet that died rich. Make one fortune, you're sure to blow it in trying to find another. I'm no exception to the rule..."
~Treasure of the Sierra Madre (1948)
Treasure of the Sierra Madre ranks among the best movies on speculation and its damaging effect on the psychology of men. And there-in lie lessons for gold investors.
In 2009 and 2010 virtually every investment conference I attended included a speaker extolling the virtues of diversifying a portfolio into gold. That advice went over well as the price of gold rose. But gold has declined 25% from its 2011 peak, especially as the market experienced a rush to the exits with a 10% drop over the past 2 weeks.
Events of the past 2 weeks, including an emerging consensus that gold is over-valued, triggered a panic in the gold market. First, two weeks ago Goldman Sachs formally reversed a bullish forecast for gold. Then a rumor spread that Cyprus was dumping its 14 tons of gold reserves onto the market. Paul Krugman wrote an article summarizing the evidence that there has been unsustainable investment in gold (Lust for Gold). Krugman cites a 2011 Gallup poll in which one-third of Americans called gold the best long-term investment. Barry Ritholtz debunks some of the cult-like rationalizations of gold hoarders in this interview. The gold consensus has rapidly turned bearish.
Back to Treasure of the Sierra Madre (1948):
Howard: Gold itself ain't good for nothin' except makin' jewelry with and gold teeth. Aw, gold's a devilish sort of a thing anyway. You start out to tell yourself you'll be satisfied with twenty-five thousand handsome smackers worth of it, 'so help me Lord and cross my heart.' Fine resolution. ... Ten you want to get twenty-five. Twenty-five you want to get fifty. Fifty, a hundred. Like roulette. One more turn, you know, always one more.
The historical lust for gold has affected all of us, in ways obvious and subtle. To give one example, Latin America was permanently shaped by the Spanish search for gold. Conquistadors such as Coronado spent their family fortunes searching for Cibola - the lost city of gold - from Patagonia to as far north as the plains of present-day Kansas. In fact, a high school in my home town was named after Coronado. Gold has an allure that drives people to fantasy and wild speculation - often to the point of ruin. And the psychology that drives its pursuit is common across the human treatment of all similar assets.
In this week's newsletter we take a look at gold, the psychology driving its prices, make a few forecasts, and revisit crypto-currencies.
April 20, 2013
Cryptocurrencies, Bitcoin, and the Psychology Driving Global Currency Values
What is Value?
"There is no subtler, no surer means of overturning the existing basis of society than to debauch the currency. The process engages all the hidden forces of economic law on the side of destruction, and does it in a manner which not one man in a million is able to diagnose."
~ John Maynard Keynes, "The Economic Consequences of the Peace" (1919). In 2008 MarketPsych's CTO Thomas Hartman worked on a secretive software project in Panama. The goal of that project was to disrupt and supplant the global banking system. The fruits of such efforts are emerging in the increasing importance and acceptance of cryptocurrencies. Last week Eurogroup chief Jeroen Dijsselbloem revealed that the Cypriot bank bailout package is a template for future euro rescues. European bank depositors can expect seizures of uninsured deposits if their bank becomes insolvent. In Cyprus today, bank account holders are limited to a withdrawals of 300 Euro daily or up to 5,000 Euro monthly. The freeze of Euro-denominated bank accounts in Cyprus corresponded with a spike in the price of gold and a massive bull run in the value of cryptocurrencies such as Bitcoin, LiteCoin, and Namecoin. As the realization dawns that similar seizures could happen in Portugal, Spain, and Italy, capital will continue searching for a haven away from the sticky fingers of banking and government officials. Cryptocurrencies are currencies without a government sponsor, composed of unique cryptographic codes (solutions to complex mathematics problems), and consensus legitimacy. Their conversion value to national currencies depends entirely on supply, demand, security, confidence, and the whims of the investing crowd and businesses who accept them. The most secure and popular cryptocurrency is Bitcoin. Bitcoin was released in 2009 as the financial crisis smoldered, and there is now over $1 billion of Bitcoin in circulation today and hundreds of legitimate (and many sketchy) businesses who accept it. In the first three months of 2013 Bitcoin has appreciated 6-fold versus the U.S. Dollar. Cryptocurrencies are benefitting from the loss of public trust in fiat currencies issued by currency war-prone governments. We are in a unique position to comment on cryptocurrencies and the psychology of currency valuations. For one, our software team includes early crypto-currency speculators and of course our very own CTO Thomas - an evangelist in the development of alternative currencies.
Secondly, our psychological data is the world's largest database of currency-related sentiment, and we have been mining that data for currency predictive models. And thirdly, and unfortunately, one of our team members is a Russian living in Cyprus who is frozen out of his Cypriot bank account. Needless to say, this week's newsletter strikes close to home. This week we'll take a look at the amazing rise of the world's first viable cryptocurrencies. Then we briefly review our research on the psychological drivers of currency valuation, and we touch on a future where currencies are no longer solely under the control of governmental and banking officials.
March 30, 2013
New Highs! Stock Promoters, Media Manipulation, and Timing Price Reversals
Pump and Dump
We've had a busy 6 weeks since our last newsletter, and we have many new discoveries to write about. As U.S. equity markets reach new highs, today's newsletter looks at a key factor that both drives poor trading behavior and creates profitable patterns in the markets: price forecasts in the media. First we'll start with a look at a primal, but instructive, form of influence over market prices - the pump-and-dump in social media. Then, by quantifying and backtesting media price forecasts using the Thomson Reuters MarketPsych Indices, we see that investors who follow predictions in both the news and social media lose significant amounts of money. Inversely, those who play against the media do much better.
____
Two days before receiving a subpoena from the SEC, 15 year-old Jonathan Lebed posted the following pitch 200 separate times on Yahoo! Finance stock message boards. He was promoting the stock of a company called Firetector (ticker symbol FTEC):
Subj: THE MOST UNDERVALUED STOCK EVER
Date: 2/03/00 3:43 pm Pacific Standard Time
From: LebedTG1
FTEC is starting to break out! Next week, this thing will EXPLODE. ...
Currently FTEC is trading for just $2 1/2! I am expecting to see FTEC at $20 VERY SOON....
According to Michael Lewis, author of Liar's Poker and Moneyball, Lebed gradually honed the appeal of his promotional messages. Through a trial-and-error process, Lebed learned which aspects of his stock appeals drove investors to buy in spite of any conscious misgivings.
In a 2001 press release, the SEC accused Lebed of touting stocks so that he could make a quick profit on the price jumps he himself had engineered:
On eleven separate occasions between August 23, 1999 (when Lebed was 14 years old) and February 4, 2000, Lebed, of Cedar Grove, New Jersey, engaged in a scheme on the Internet in which he purchased, through brokerage accounts, a large block of a thinly-traded microcap stock. Within hours of making the purchase, Lebed sent numerous false and/or misleading unsolicited e-mail messages, or "spam," primarily to various Yahoo! Finance message boards, touting the stock he had just purchased. Lebed then sold all of these shares, usually within 24 hours, profiting from the increase in price his messages had caused.
Jonathan Lebed settled out of court, reportedly for a minor fine, and kept the bulk of his trading profits. He continued operating as a paid stock promoter through the 2000s at lebed.biz.
When Lebed predicted an explosive gain in a stock price, readers experienced a surge of dopamine. The dopamine increased motivation to act, bolstered confidence, and led investors to discard rational analysis. Interestingly, this is a similar strategy to that employed by the ex-wife (and assorted family members) of late Nigerian President Sani Abacha. You may have received emails allegedly from them offering you a multimillion dollar payout if you agree to hide their billion dollar fortune in your personal bank account (a.k.a. the Nigerian 419 scam). The vast size of the reward blinds gullible respondents to its absurdity.
March 15, 2013
Buy to the Sound of Cannons: The Relationship Between Global Violence and Asset Prices
Global Violence and Fear
"Buy on the sound of cannons, sell on the sound of trumpets" ~ Nathan Rothschild, 1810
Maybe you haven't heard the above quote, but you've certainly heard this variation with the same meaning - "buy on fear and sell on greed."
Easy to say, hard to do. It is far easier to speak those words in a sedate lecture hall than it is to execute on them during a civil war.
Fear is one of our oldest emotions. First we must nourish ourselves to live. Second we must avoid danger in order to survive. The biological fear response saturates our bodies and brains with hormones and neurotransmitters and throws our rational prefrontal cortex offline. In the midst of fear, our breathing, heart rate, circulation, muscle tone, digestion, and sensory interpretations all change. As our fear prepares us for fight, flight, or freezing, it creates an involuntary physiological response that we have little conscious control over. Fear turns our bodies into unthinking survival machines.
I like the cannons in the opening quote because the fear that war and violence cause are significant to our predictive models for countries and currencies. It is difficult to measure expressions of fear in the news - reporters are trained not to express their own emotions. But they can report on the images and interviews that stir fear in them, and those interviews are usually around the topics of violence, chaos, and social breakdown.
Last newsletter we introduced the idea of buying stocks in countries with the greatest government instability as reported in the news flow. In this newsletter we introduce a rotating model of global equity investing. First we describe our automatically updating global investment risk map. It is a counterintuitive fact that but the least risky countries for investing are those that are perceived to have the most risk - the risk is already priced in. Then towards the end of the newsletter we identify the sentiments that are most influential over currency prices.
We'll be in San Francisco on February 13th for a company meeting. If you're interested in learning more about our company, please get in touch.
February 03, 2013
A Guide to International Investing: Unstable Government? Buy it. Happy People? Short it.
Heart of Darkness
"He has to live in the midst of the incomprehensible, which is detestable. And it has a fascination, too, which goes to work upon him. The fascination of the abomination--you know. Imagine the growing regrets, the longing to escape, the powerless disgust, the surrender, the hate."
- Joseph Conrad, Heart of Darkness
When a government fails - whether due to warfare, failure to provide services, or insolvency and cutbacks - the citizens of the country go through several psychological stages.
Unfortunately I've witnessed these stages, and gone through them myself. I used to enjoy off-the-beaten path travel and journeyed to places in transition such as post-Soviet Central Asia in 1992 and Central Africa in 1996 (inspiration for the Heart of Darkness quote above and throughout today's letter). The particulars of my experiences are too lengthy and surreal to write about here, but suffice it to say, when the lights go off, the bugs come out.
When a government first falls - when the President flees the country or a brand new group enters power - there is at first hope that business will continue as usual. This represents the stage of denial. In the second stage, as laws change or law and order break down entirely, citizens enter a stage of paralysis. I saw this in the post office in Kisangani, Zaire, where well-dressed postal workers came to work every day despite not having been paid in 2 years and having no work to do. Paralyzed citizens protect valuable possessions and listen to every rumor for signs of danger. Their indecision is broken when they realize that inaction will cost them dearly, often leading to the third stage: disorganized panic and escape. They run away or, if they don't have the resources to run, they bunker down. In the fourth stage, they become accustomed to the new order, either learning to live as a refugee or accepting the new authorities and laws (or the arbitrariness of the laws).
In Africa in 1996 I traveled from Uganda to Cameroon overland. In Zaire (now Congo) the government was absent - warlords and bandits were dividing the country. And when I was in the Central African Republic, a rebel army was camped outside the capital, regrouping for a second assault. Adventurous diamond and gold traders remained in Zaire, despite the danger, buying up gold nuggets and uncut diamonds at bargain-basement prices from local miners. Nothing good came from those African failed states, which remain troubled today, but those hardy entrepreneurs profited handsomely - if they survived.
January 19, 2013
Making Resolutions Stick and The Least Trusted Banks of 2012
Resolutions, Honestly
"New Year's Day now is the accepted time to make your regular annual good resolutions. Next week you can begin paving hell with them as usual"
~ Mark Twain
It's 2013. Lot of resolutions have been made: "this year I'll exercise more, eat better, be more patient, etc..." Yet as behavioral economists we know that most resolutions won't be kept. Those resolutions that are honored are usually simple and straightforward - goals that would have been achieved even if not articulated.
In my experience behavioral economists are some of the worst resolution-keepers. I won't cast stones, as I'm in a glass house of my own construction. Remember our weekly newsletter? That's right, the one that comes every two weeks? Or sometimes every four weeks?... Despite heroic weekly efforts, we're not weekly newsletter goal-achievers.
So we set about to get it right this year. Why is the resolution failure rate so high, even by "professionals" who design behavioral guides like this one? What can we honestly do to make sure our newsletter is published weekly? As we dug into this, we realized we had entered a vault in the collective unconscious.
Resolutions aren't what we think they are.
January 04, 2013
2012
The Happiest Day of the Year! and China Sentiment
The Happiest Day
"During holidays, [Norman Vincent] Peale once suggested, you should make 'a deliberate effort to speak hopefully about everything.'"
~ Oliver Burkeman
Our in-house psychologist - Dr. Frank Murtha - recently asked me, "You've got all this data on emotions so tell me, what's the Happiest Day of the year? And as a matter of fact, what's the Angriest Day? And the Most Pessimistic Day?"
I had no idea. Based on work by Aleksander Fafula, our data genius, we know the happiest month is January (when we control for holiday greetings) and the Angriest month is September. But days? We hadn't thought to look.
To investigate, we ran an analysis using the Thomson Reuters MarketPsych Indices social media-based sentiment indices for the S&P 500. These indices cover every year from 1998 through November 2012.
What did we find? First of all, and fortunately, the next few weeks don't contain extremely Angry or Pessimistic days. The next 3 weeks do, however, contain the Happiest and the Most Optimistic Days. Norman Vincent Peale's advice has possibly been taken to heart, but perhaps more for unconscious biological reasons (seasonal neurochemical and hormonal shifts) rather than people consciously taking Peale's words to heart (the advice itself is useless).
Interestingly, over periods of weeks we see clusters of days or weeks being generally of the same emotional state. When we look at weekly patterns alone, we see that investors express the most Joy and Optimism in the first week of January (approximately 3rd through 6th), with the second week of January close behind. Also important, Gloom, Anger, and Fear levels are the lowest during those weeks - not only are positive emotions high, but negative emotions are low. In summary, investors are generally happiest at the beginning of the year, when enjoying a fresh start (perhaps this has something to do with the January Effect in stock markets)
For specific days, the 23rd of December is the Most Joyful (today!!!), and January 9th is the Most Optimistic. Note that market holidays are excluded from the analysis.
So... we hope you have been enjoying Financial Happiness Day today!
In honor of Financial Happiness Day (and the Fiscal Cliff), check out Dr. Murtha's zany video spoofing the struggles of financial advisors around the fiscal cliff with our old friends, Bob (an individual investor) and his financial advisor Helen.
But now on to more serious matters...
December 22, 2012
The Psychology of the Fiscal Cliff, Bullying, and the Impact of Stress on Markets.
Prison Yard Dominance
"You have to remember one thing about the will of the people: it wasn't that long ago that we were swept away by the Macarena."
- Jon Stewart
Leading group therapy for mentally ill prisoners is never boring. But is it dangerous? Not really. Humorous? Yes, sometimes. Enlightening? Nearly always. In one fascinating group, a mutiny against my leadership was led by a psychologically saavy gang-leader.
I bring up this experience because it mirrors the fiscal cliff drama playing out in Washington. While I was confronted by a different league of bully in prison, the techniques I used to regain control of the group may be used in Washington to restore legislative momentum, and if we see them emerge, we will know compromise is near.
The mutiny in my group occurred minutes into our first group therapy event of the season. Before we were more than two minutes into introductions, an intense young man stood up, look straight into my eyes, squared off before me, and loudly informed the group: "He's just some rich kid doctor from Santa Monica. He doesn't know %$#&@ about what we've been through!"
I looked at him, knocked back by his outburst. I protested, "No that's not right..."
He went on, "Let's go guys, we don't need this, we're done with this &$#*&@." He walked out with his fellow group members, staring me down as they filed out of the room.
I was left alone, embarrassed and befuddled. After all, I was in fact a well-to-do young doctor from Santa Monica, and the fact that he - a powerful gang leader with an outside network - knew about my personal life was truly disturbing. I needed professional advice about how to handle a situation like that one, so I turned to a wise mentor.
December 08, 2012
Slugs and Sugar: Gender Effects on Investing and Nokia
Puppy Dog Tails
Slugs and snails
And puppy-dogs' tails, That's what little boys are made of. Sugar and spice And everything nice, That's what little girls are made of.
- Nineteenth century English nursery rhyme.
_____________________________________________________
"Honey, what happened to our retirement savings?"
If you're a man, your partner may have asked you this at some point in the past few years.
"Hmmm, I'm not sure what you mean?" you may evasively answer. (The truth is, you're very aware that you invested too much in that awesome small cap tech stock. Sure it's down 50% since you bought in, but it is such a GREAT story, it HAS to work out.)
She persists, "I mean, how come the account has less money than last year? The market was up this year."
Now if you're like most men, you want to please your wife (and yourself). And you probably believe what you say next: "Um, well, I invested in CyberNanoTech and they had a bit of a setback this year because of the FDA, but their long term prospects are amazing, everyone says so, so I'm holding tight."
"What is CyberMamoSpec?"
"Well, Joey at the Club was saying they have this world changing...."
"Honey, what does Joey know about investing, isn't he an orthodontist?"
This is why men need wives, to pop their unreality bubbles.
It's at this point in the conversation that his wife may pick up the phone to call a financial advisor. And if all goes according to her plan, which is likely, their financial relationship dynamics will become the domain of a financial advisor, which is another story (and one I often speak to advisors about).
November 23, 2012
Traders Who Take Sex Hormones and 'Roid Rage in Markets
Hormones in the Markets
"If taking female hormones actually helped you do your job, they would simply hire women here...But they don't. They don't think women are aggressive enough."
~ New York Post, citing an SAC employee and reprinted here.
A couple years ago I began coaching a trader with a hormonal problem.
He told me, "When I trade at my best I'm aggressive and decisive. Ready for whatever the market throws at me."
"And how have you been trading lately?" I asked.
"I'm none of those. I'm indecisive and cautious. I'm just sort of limp when I need to be strong and fast. And I just keep losing money. You know, it's as if I've lost my mojo."
November 17, 2012
Hurricane Psychology, Buying Pessimism (RIMM), and Finding Redemption
Man Problems
While forecasters predicted Hurricane Sandy would be the worst storm in New York history, many people did not prepare adequately.
OK, to be perfectly honest, I did not prepare adequately. In response to a question posed by my New England-born wife before the storm, "Shouldn't we buy a generator?" My response, "Generator? Who needs a generator?" will probably rank as one of the more egregious on my Clueless-Man-Responses List, just ahead of #6 "No, I know where I'm going, I don't need a map," and just behind #4 "Anniversary? You mean today?"
In my (and my wife's) quest to understand the Clueless-Man-Responses List and why it continues to be populated, I did a bit of research. Turns out that due to a mental mechanism called the "projection bias," people's intellectual assessments of a danger ("worst storm ever") conflict with their emotional experience ("I'm fine right now, those forecasters must be exaggerating.") In these scenarios, most people go with their gut - "It'll be fine, it always is." As a result, there is inertia in their preparations.
In fact, as I write this letter I am warm and cozy (and a tad humiliated) at my wife's parents' house in Maine. A couple days without heat and power was enough to turn us into storm refugees. Thank goodness for the kindness we've encountered since the storm. One thing I was impressed by after the storm was the tremendous goodwill and comraderie of neighbors in affected communities.
November 03, 2012
Resentment Investing: Fading Soros, Emotional Ruts, and Doubting the Bull
Breaking the Bank
When George Soros "broke the Bank of England," famously earning him $1.1 billion on a single trade, it was not only the Bank of England who was rocked by losses. Some ambitious and formerly successful traders had bet against Soros - traders such as Bob.
Some background: In 1990 the Britain's Thatcher government decided to join the European Exchange Rate Mechanism (ERM). The ERM was a system to reduce exchange-rate variability. With U.K. inflation at three times the rate of Germany's and interest rates at 15 percent, the conditions for remaining the ERM were not favorable.
Due to its inherent impossibility, as well as short-selling by an international group of currency speculators led by George Soros, the UK was forced to withdraw from the ERM on September 16, 1992 ("Black Wednesday"). Soros' trade was virtually guaranteed to be successful due to the large interest rate differentials between the U.K. and Germany. Not all central bankers learned the lesson - Soros and others profited from similar trades put on before the Asian Currency Crisis of 1997.
October 26, 2012
Psychopaths at Hedge Funds, Google, and the Persistence of Bad News
The School of Life
Street craps - played with two dice on a sidewalk - is little like its casino brother. The odds are different, the pace is much faster, and your dealer is typically a hustler or worse, a psychopath. As for me, I learned how to deal street craps in prison.
As a psychiatrist I've evaluated competency and treated mental illness among prisoners, some of whom were hustlers or psychopaths. Street hustlers in my experience are usually quite engaging and reflective, able to gain insights and do productive psychological work. Psychopaths are cold and heartless - mimicking the emotions and social styles they have seen expressed by others. Typically psychopaths don't see a psychiatrist voluntarily, and when they do, they spend a good deal of time looking for angles - how to game the system and get what they want from the doctor.
When a psychopath offered to teach me street craps in a prison, I knew from my clinical training that he was being friendly only because he wanted something from me - information, medications (some medications are used as currency in prisons), or to learn some therapeutic tricks he could use on others. Nonetheless I was riveted as he explicitly taught me how to spin the dice to get the numbers I wanted and how to tap and blow on them to distract unsuspecting clients. At the same time, I told myself, I was gaining valuable insight into the mind of a psychopath. After the tutorial -- and alas too late to do anything about it -- I realized to my horror that my pen was missing.
He wasn't interested in passing time with some friendly banter or in gaining some psychological insights while he was teaching me how to roll the dice - the dice lesson was for distracting me while he swiped my pen.
October 19, 2012
Toxic Optimism: The Dutch-French Disease, Facebook Face-Plant, and How to Profit from Delusion
Optimism, Bind Optimism, and Balance
"The intelligent investor is a realist who sells to optimists and buys from pessimists."
~ Benjamin Graham, Jason Zweig in The Intelligent Investor, Rev. Ed.
As a psychiatrist I've born witness to many extremes of mood. I've tried to steer manic venture capitalists away from spending their life savings on cocaine and sports cars. And on the other extreme I've born witness to the deep pessimism and despair of suicidal clients, trying to help them choose life over oblivion. My efforts are not always successful, and I've learned a lot about the limits of rationality when faced with extremes of mania and despair. At both extremes many of the people I've worked with are highly intelligent and believe they are acting with perfect rationality. Yet despite their innate intelligence, deeper emotions occasionally sweep away their anchor to reality.
In Ben Graham's quote above, one could be mistaken for thinking of the "intelligent investor" as a robot or algorithm. Human investors feel the extreme optimism and pessimism of the markets. And the effect of this optimism and pessimism is, almost universally, to bias their interpretations of real events. While the best investors feel the moods of the market (that is, they are empathic), they also have the almost super-human ability to act contrary to their emotional biases. Graham's vision of intelligence in the 1950s is what we today call emotional intelligence.
Warren Buffett called Graham's book, The Intelligent Investor, "By far the best book on investing ever written." I think it's worth looking for lessons in Graham's thoughts on optimism.
Today's letter will examine the nature of optimism in the financial markets: How we experience it, how it distorts our investing, how it plays out in markets, and how we can succeed while being both optimistic AND realistic. As we go through these themes, we will look at French and Dutch optimism in the Euro-zone, the delusional optimism at the launch of the Facebook IPO (see Dr. Murtha's hilarious Part 2 video), how portfolio managers can use optimism to their advantage, and visit some tools and techniques for making ourselves better investors.
But first, some housekeeping...
August 14, 2012
Greece, Spain, and the Psychology of Financial Collapse
Collapse of Dreams
"[T]he values to which people cling most stubbornly under inappropriate conditions are those values that were previously the source of their greatest triumphs."
- Jared Diamond, Collapse: How Societies Choose to Fail or SucceedIn Collapse: How Societies Choose to Fail or Succeed, Jared Diamond (UCLA professor of Geography) described the collapse of civilizations as a gradual environmental process, fueled by systematic behavioral weaknesses evident in inertia and short-term thinking, culminating in a rapid unwinding too rapid to stop. As he describes it, as environmental stress increased in prior failing societies, social and economic disparities widened. Rather than looking to identify empirical causes and create functional solutions to save themselves, these societies fractured along political and class lines. As stress increased, the various factions hardened their tactics, ultimately physically attacking internal political enemies and igniting civil wars which destroyed the social and cultural mores that upheld institutions.
I was reminded of Diamond's thesis a few weeks ago in Canada, of all places. I was in Toronto's financial district when I was handed, with a direct and sincere look, a manifesto on the evils of capitalism.
Now, when I lived in San Francisco and Austin (two cities I love), I often received such manifestos - usually followed by some unsolicited recommendations regarding marijuana cultivation or freeing Tibet. It was all fairly benign. But the Toronto pamphleteer was not unhinged, malodorous, or intoxicated - he was sincere, healthy, and appeared overtly rational. His apparent reasonableness shocked me more than the manifesto itself: A reasonable man pushing an overthrow of capitalism. That same night, student riots in Montreal over tuition increases led to the arrest of 122.
Democratic governments in the developed world have borrowed money to finance their promises. Without displaying adequate performance. As a result, voters are disappointed and angry, and now inclined to try extreme alternatives.
While I'll discuss social collapse in light of Greece this week, I think we should also take this opportunity to look at our own societies. The unraveling has already begun. What can we do to prevent a similar meltdown?
I consider myself optimistic, so it pains me to return to the topic of collapse. That said, I'm of the opinion that facing an ugly truth sooner allows us to have a happier life later. Sadly politicians have the incentive to avoid hard choices in the short term, and as a result the pain of collapse will be that much greater.
New public policies that account for human (and elected official) psychological weaknesses are desperately needed. Fortunately, such an effort has begun. Read on to learn more.
But first, some housekeeping...
June 11, 2012
Regret, When to Sell AAPL, and Timing Bubble Tops
Shorting Bubbles - Ouch!
I've made many unfortunate investments over the years, but one in particular still bothers me. In 1998 it was very clear that the technology sector was in the midst of a historic bubble. There were many overvalued stocks - with no earnings, high debt, too-good-to-be-true stories, and extremely high valuations. I picked Amazon.com due to its high level of hype, and I sold short some shares.
A few months later I had lost 40% on that investment. Then a few months after that 80%. By the time I had lost 120%, I bought back my shares. (And good thing I did, the stock continued much higher before it finally peaked). I learned a lesson best-articulated by John Maynard Keynes: "Markets can remain irrational a lot longer than you and I can remain solvent."
In the post-mortem analysis of that investment I looked back on my thought process. My conviction had been high, I knew I was right. Diagnosis: Overconfidence. And as a result of overconfidence, a weak risk-management plan. This trade changed how I think about trading timing and risk management. (It's ironic how improving risk management always becomes urgent AFTER a big loss).
Steamrolled by the crowd, and my lesson learned, I turned to the study of timing bubbles. I wondered, how could I time the expansion and ultimate collapse of market sentiment around hot tech stocks like Amazon.com?
I decided to start by looking inward - what were investors in Amazon thinking (or not thinking) when they bought in? And what triggered the ultimate fall in the price? In fact, my senior research project in medical school was on "The Social Psychology of Bubbles and Panics" (I was extremely privileged to work with a pre-eminent researcher on impulse-control - Ernest Barratt, Ph.D.).
It turned out that expectations are key to bubbles, and expectations are rooted in the brain's emotional circuitry. That circuitry is easily activated by extraneous events. In fact, researchers have found that completely unrelated positive events (movie clips, pictures of happy faces, pornography) prime us toward taking excessive financial risk (Read more in today's Researcher's Corner below).
We've had a similar sort of timing problem the past several newsletters. And from our research, we know that a key success trait of investors is good timing - rapid execution on high conviction ideas. Our monthly newsletter takes about 10 days from conception to publication, and during that 10 days our forecasts often become stale, rendering the monthly recommendations less profitable (e.g., the oil and copper short hints last month worked out a few days before publication - fortunately the decline in oil and copper prices continues).
Before we jump into how to time bubbles, first some housekeeping.
April 09, 2012
Middle Eastern Paranoia, Oil, and the Psychology of Playing Chicken
Shopping Carts and Playing Chicken
When I was a boy in Texas the neighborhood children enjoyed playing chicken, usually with speeding shopping carts. Two children were pilots and two children were bombs. The pilots dutifully ran their bombs up to full speed, released them, and ... CRASH. Ideally your shopping cart remained standing after the collision, in which case you won. Fortunately, two shopping carts crashing sounds much more painful than it actually was.
But this newsletter isn't about bored suburban kids.
This month we look at a much more deadly game of chicken between Iran and Israel (and by proxy, the U.S.A.). Mirroring the escalating tensions, oil prices are up over 10% in the past five weeks. Higher oil and gasoline prices are already impairing our economic recovery.
This newsletter will examine Behavioral Game Theory as it applies to Iran and Israel. What is the best strategy to deter a foe who may be crazy, short of war? We will then look at the current sentiment snapshot of news and social media as it relates to Oil, Iran and Israel, which reveals some surprises about global mood. The psychology behind Oil's recent price rise reveals whether the rally is sustainable (hint: fear is rarely sustainable).
In addition to our usual haunts of New York and Los Angeles, we will be speaking in San Antonio, western Pennsylvania, a CFA webinar, and San Francisco in March - we're look forward to catching up with our friends in those cities!
March 10, 2012